This is the multi-page printable view of this section. Click here to print.
Quick Start
1 - HugeGraph-Server Quick Start
1 HugeGraph-Server 概述
HugeGraph-Server 是 HugeGraph 项目的核心部分,包含 graph-core、backend、API 等子模块。
Core 模块是 Tinkerpop 接口的实现,Backend 模块用于管理数据存储,目前支持的后端包括:Memory、Cassandra、ScyllaDB 以及 RocksDB,API 模块提供 HTTP Server,将 Client 的 HTTP 请求转化为对 Core 的调用。
文档中会出现
HugeGraph-Server
及HugeGraphServer
这两种写法,其他组件也类似。 这两种写法含义上并明显差异,可以这么区分:HugeGraph-Server
表示服务端相关组件代码,HugeGraphServer
表示服务进程。
2 依赖
2.1 安装 Java 11 (JDK 11)
请优先考虑在 Java 11 的环境上启动 HugeGraph-Server
(在 1.5.0 版前,会保留对 Java 8 的基本兼容)
在往下阅读之前先执行 java -version
命令确认 jdk 版本
注:使用 Java 8 启动 HugeGraph-Server 会失去一些安全性的保障,也会降低性能相关指标
3 部署
有四种方式可以部署 HugeGraph-Server 组件:
- 方式 1:使用 Docker 容器 (便于测试)
- 方式 2:下载 tar 包
- 方式 3:源码编译
- 方式 4:使用 tools 工具部署 (Outdated)
注意 生产或对外网暴露访问的环境必须使用 Java 11 并开启 Auth 权限认证, 否则会有安全隐患。
3.1 使用 Docker 容器 (便于测试)
可参考 Docker 部署方式。
我们可以使用 docker run -itd --name=server -p 8080:8080 hugegraph/hugegraph:1.5.0
去快速启动一个内置了 RocksDB
的 Hugegraph server
.
可选项:
- 可以使用
docker exec -it server bash
进入容器完成一些操作 - 可以使用
docker run -itd --name=server -p 8080:8080 -e PRELOAD="true" hugegraph/hugegraph:1.5.0
在启动的时候预加载一个内置的样例图。可以通过RESTful API
进行验证。具体步骤可以参考 5.1.1 - 可以使用
-e PASSWORD=123456
设置是否开启鉴权模式以及 admin 的密码,具体步骤可以参考 Config Authentication
如果使用 docker desktop,则可以按照如下的方式设置可选项:
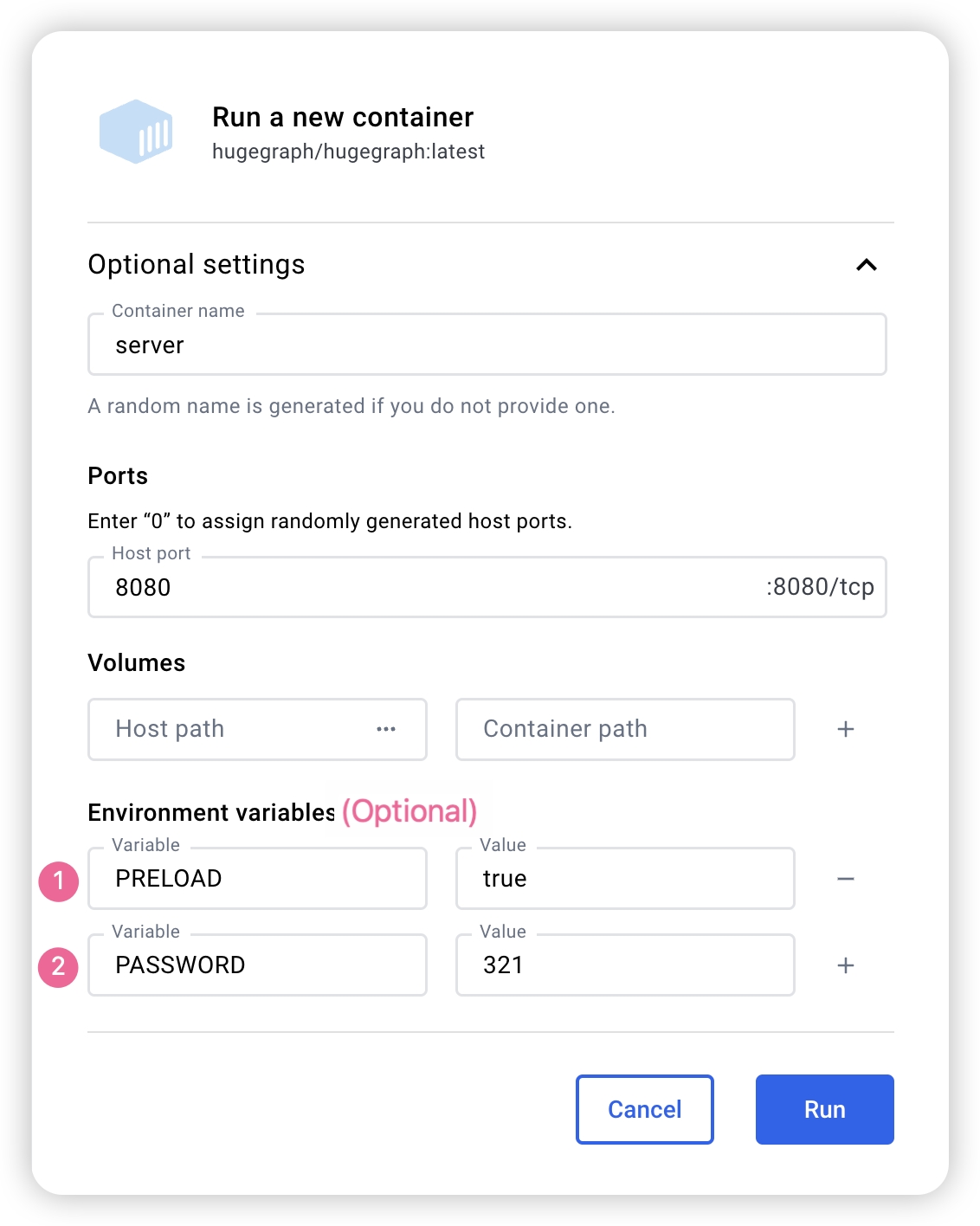
另外,如果我们希望能够在一个文件中管理除了 server
之外的其他 Hugegraph 相关的实例,我们也可以使用 docker-compose
完成部署,使用命令 docker-compose up -d
,(当然只配置 server
也是可以的)以下是一个样例的 docker-compose.yml
:
version: '3'
services:
server:
image: hugegraph/hugegraph:1.5.0
container_name: server
# environment:
# - PRELOAD=true 为可选参数,为 True 时可以在启动的时候预加载一个内置的样例图
# - PASSWORD=123456 为可选参数,设置的时候可以开启鉴权模式,并设置密码
ports:
- 8080:8080
注意:
hugegraph 的 docker 镜像是一个便捷版本,用于快速启动 hugegraph,并不是官方发布物料包方式。你可以从 ASF Release Distribution Policy 中得到更多细节。
推荐使用
release tag
(如1.5.0/1.x.0
) 以获取稳定版。使用latest
tag 可以使用开发中的最新功能。
3.2 下载 tar 包
# use the latest version, here is 1.5.0 for example
wget https://downloads.apache.org/incubator/hugegraph/{version}/apache-hugegraph-incubating-{version}.tar.gz
tar zxf *hugegraph*.tar.gz
3.3 源码编译
源码编译前请确保本机有安装 wget/curl
命令
下载 HugeGraph 源代码
git clone https://github.com/apache/hugegraph.git
编译打包生成 tar 包
cd hugegraph
# (Optional) use "-P stage" param if you build failed with the latest code(during pre-release period)
mvn package -DskipTests
执行日志如下:
......
[INFO] Reactor Summary for hugegraph 1.5.0:
[INFO]
[INFO] hugegraph .......................................... SUCCESS [ 2.405 s]
[INFO] hugegraph-core ..................................... SUCCESS [ 13.405 s]
[INFO] hugegraph-api ...................................... SUCCESS [ 25.943 s]
[INFO] hugegraph-cassandra ................................ SUCCESS [ 54.270 s]
[INFO] hugegraph-scylladb ................................. SUCCESS [ 1.032 s]
[INFO] hugegraph-rocksdb .................................. SUCCESS [ 34.752 s]
[INFO] hugegraph-mysql .................................... SUCCESS [ 1.778 s]
[INFO] hugegraph-palo ..................................... SUCCESS [ 1.070 s]
[INFO] hugegraph-hbase .................................... SUCCESS [ 32.124 s]
[INFO] hugegraph-postgresql ............................... SUCCESS [ 1.823 s]
[INFO] hugegraph-dist ..................................... SUCCESS [ 17.426 s]
[INFO] hugegraph-example .................................. SUCCESS [ 1.941 s]
[INFO] hugegraph-test ..................................... SUCCESS [01:01 min]
[INFO] ------------------------------------------------------------------------
[INFO] BUILD SUCCESS
[INFO] ------------------------------------------------------------------------
......
执行成功后,在 hugegraph 目录下生成 *hugegraph-*.tar.gz
文件,就是编译生成的 tar 包。
过时的 tools 工具安装
#### 3.4 使用 tools 工具部署 (Outdated)
HugeGraph-Tools 提供了一键部署的命令行工具,用户可以使用该工具快速地一键下载、解压、配置并启动 HugeGraph-Server 和 HugeGraph-Hubble,最新的 HugeGraph-Toolchain 中已经包含所有的这些工具,直接下载它解压就有工具包集合了
```bash
# download toolchain package, it includes loader + tool + hubble, please check the latest version (here is 1.5.0)
wget https://downloads.apache.org/incubator/hugegraph/1.5.0/apache-hugegraph-toolchain-incubating-1.5.0.tar.gz
tar zxf *hugegraph-*.tar.gz
# enter the tool's package
cd *hugegraph*/*tool*
注:
${version}
为版本号,最新版本号可参考 Download 页面,或直接从 Download 页面点击链接下载
HugeGraph-Tools 的总入口脚本是 bin/hugegraph
,用户可以使用 help
子命令查看其用法,这里只介绍一键部署的命令。
bin/hugegraph deploy -v {hugegraph-version} -p {install-path} [-u {download-path-prefix}]
{hugegraph-version}
表示要部署的 HugeGraphServer 及 HugeGraphStudio 的版本,用户可查看 conf/version-mapping.yaml
文件获取版本信息,{install-path}
指定 HugeGraphServer 及 HugeGraphStudio 的安装目录,{download-path-prefix}
可选,指定 HugeGraphServer 及 HugeGraphStudio tar 包的下载地址,不提供时使用默认下载地址,比如要启动 0.6 版本的 HugeGraph-Server 及 HugeGraphStudio 将上述命令写为 bin/hugegraph deploy -v 0.6 -p services
即可。
4 配置
如果需要快速启动 HugeGraph 仅用于测试,那么只需要进行少数几个配置项的修改即可(见下一节)。
5 启动
5.1 使用启动脚本启动
启动分为"首次启动"和"非首次启动",这么区分是因为在第一次启动前需要初始化后端数据库,然后启动服务。
而在人为停掉服务后,或者其他原因需要再次启动服务时,因为后端数据库是持久化存在的,直接启动服务即可。
HugeGraphServer 启动时会连接后端存储并尝试检查后端存储版本号,如果未初始化后端或者后端已初始化但版本不匹配时(旧版本数据),HugeGraphServer 会启动失败,并给出错误信息。
如果需要外部访问 HugeGraphServer,请修改 rest-server.properties
的 restserver.url
配置项(默认为 http://127.0.0.1:8080
),修改成机器名或 IP 地址。
由于各种后端所需的配置(hugegraph.properties)及启动步骤略有不同,下面逐一对各后端的配置及启动做介绍。
注: 如果想要开启 HugeGraph 权限系统,在启动 Server 之前应按照 Server 鉴权配置 进行配置。(尤其是生产环境/外网环境须开启)
5.1.1 RocksDB
点击展开/折叠 RocksDB 配置及启动方法
RocksDB 是一个嵌入式的数据库,不需要手动安装部署,要求 GCC 版本 >= 4.3.0(GLIBCXX_3.4.10),如不满足,需要提前升级 GCC
修改 hugegraph.properties
backend=rocksdb
serializer=binary
rocksdb.data_path=.
rocksdb.wal_path=.
初始化数据库(第一次启动时或在 conf/graphs/
下手动添加了新配置时需要进行初始化)
cd *hugegraph-${version}
bin/init-store.sh
启动 server
bin/start-hugegraph.sh
Starting HugeGraphServer...
Connecting to HugeGraphServer (http://127.0.0.1:8080/graphs)....OK
提示的 url 与 rest-server.properties
中配置的 restserver.url
一致
5.1.2 HBase
点击展开/折叠 HBase 配置及启动方法
用户需自行安装 HBase,要求版本 2.0 以上,下载地址
修改 hugegraph.properties
backend=hbase
serializer=hbase
# hbase backend config
hbase.hosts=localhost
hbase.port=2181
# Note: recommend to modify the HBase partition number by the actual/env data amount & RS amount before init store
# it may influence the loading speed a lot
#hbase.enable_partition=true
#hbase.vertex_partitions=10
#hbase.edge_partitions=30
初始化数据库(第一次启动时或在 conf/graphs/
下手动添加了新配置时需要进行初始化)
cd *hugegraph-${version}
bin/init-store.sh
启动 server
bin/start-hugegraph.sh
Starting HugeGraphServer...
Connecting to HugeGraphServer (http://127.0.0.1:8080/graphs)....OK
更多其它后端配置可参考配置项介绍
5.1.3 MySQL
点击展开/折叠 MySQL 配置及启动方法
由于 MySQL 是在 GPL 协议下,与 Apache 协议不兼容,用户需自行安装 MySQL,下载地址
下载 MySQL 的驱动包,比如 mysql-connector-java-8.0.30.jar
,并放入 HugeGraph-Server 的 lib
目录下。
修改 hugegraph.properties
,配置数据库 URL,用户名和密码,store
是数据库名,如果没有会被自动创建。
backend=mysql
serializer=mysql
store=hugegraph
# mysql backend config
jdbc.driver=com.mysql.cj.jdbc.Driver
jdbc.url=jdbc:mysql://127.0.0.1:3306
jdbc.username=
jdbc.password=
jdbc.reconnect_max_times=3
jdbc.reconnect_interval=3
jdbc.ssl_mode=false
初始化数据库(第一次启动时或在 conf/graphs/
下手动添加了新配置时需要进行初始化)
cd *hugegraph-${version}
bin/init-store.sh
启动 server
bin/start-hugegraph.sh
Starting HugeGraphServer...
Connecting to HugeGraphServer (http://127.0.0.1:8080/graphs)....OK
5.1.4 Cassandra
点击展开/折叠 Cassandra 配置及启动方法
用户需自行安装 Cassandra,要求版本 3.0 以上,下载地址
修改 hugegraph.properties
backend=cassandra
serializer=cassandra
# cassandra backend config
cassandra.host=localhost
cassandra.port=9042
cassandra.username=
cassandra.password=
#cassandra.connect_timeout=5
#cassandra.read_timeout=20
#cassandra.keyspace.strategy=SimpleStrategy
#cassandra.keyspace.replication=3
初始化数据库(第一次启动时或在 conf/graphs/
下手动添加了新配置时需要进行初始化)
cd *hugegraph-${version}
bin/init-store.sh
Initing HugeGraph Store...
2017-12-01 11:26:51 1424 [main] [INFO ] org.apache.hugegraph.HugeGraph [] - Opening backend store: 'cassandra'
2017-12-01 11:26:52 2389 [main] [INFO ] org.apache.hugegraph.backend.store.cassandra.CassandraStore [] - Failed to connect keyspace: hugegraph, try init keyspace later
2017-12-01 11:26:52 2472 [main] [INFO ] org.apache.hugegraph.backend.store.cassandra.CassandraStore [] - Failed to connect keyspace: hugegraph, try init keyspace later
2017-12-01 11:26:52 2557 [main] [INFO ] org.apache.hugegraph.backend.store.cassandra.CassandraStore [] - Failed to connect keyspace: hugegraph, try init keyspace later
2017-12-01 11:26:53 2797 [main] [INFO ] org.apache.hugegraph.backend.store.cassandra.CassandraStore [] - Store initialized: huge_graph
2017-12-01 11:26:53 2945 [main] [INFO ] org.apache.hugegraph.backend.store.cassandra.CassandraStore [] - Store initialized: huge_schema
2017-12-01 11:26:53 3044 [main] [INFO ] org.apache.hugegraph.backend.store.cassandra.CassandraStore [] - Store initialized: huge_index
2017-12-01 11:26:53 3046 [pool-3-thread-1] [INFO ] org.apache.hugegraph.backend.Transaction [] - Clear cache on event 'store.init'
2017-12-01 11:26:59 9720 [main] [INFO ] org.apache.hugegraph.HugeGraph [] - Opening backend store: 'cassandra'
2017-12-01 11:27:00 9805 [main] [INFO ] org.apache.hugegraph.backend.store.cassandra.CassandraStore [] - Failed to connect keyspace: hugegraph1, try init keyspace later
2017-12-01 11:27:00 9886 [main] [INFO ] org.apache.hugegraph.backend.store.cassandra.CassandraStore [] - Failed to connect keyspace: hugegraph1, try init keyspace later
2017-12-01 11:27:00 9955 [main] [INFO ] org.apache.hugegraph.backend.store.cassandra.CassandraStore [] - Failed to connect keyspace: hugegraph1, try init keyspace later
2017-12-01 11:27:00 10175 [main] [INFO ] org.apache.hugegraph.backend.store.cassandra.CassandraStore [] - Store initialized: huge_graph
2017-12-01 11:27:00 10321 [main] [INFO ] org.apache.hugegraph.backend.store.cassandra.CassandraStore [] - Store initialized: huge_schema
2017-12-01 11:27:00 10413 [main] [INFO ] org.apache.hugegraph.backend.store.cassandra.CassandraStore [] - Store initialized: huge_index
2017-12-01 11:27:00 10413 [pool-3-thread-1] [INFO ] org.apache.hugegraph.backend.Transaction [] - Clear cache on event 'store.init'
启动 server
bin/start-hugegraph.sh
Starting HugeGraphServer...
Connecting to HugeGraphServer (http://127.0.0.1:8080/graphs)....OK
5.1.5 Memory
点击展开/折叠 Memory 配置及启动方法
修改 hugegraph.properties
backend=memory
serializer=text
Memory 后端的数据是保存在内存中无法持久化的,不需要初始化后端,这也是唯一一个不需要初始化的后端。
启动 server
bin/start-hugegraph.sh
Starting HugeGraphServer...
Connecting to HugeGraphServer (http://127.0.0.1:8080/graphs)....OK
提示的 url 与 rest-server.properties 中配置的 restserver.url 一致
5.1.6 ScyllaDB
点击展开/折叠 ScyllaDB 配置及启动方法
用户需自行安装 ScyllaDB,推荐版本 2.1 以上,下载地址
修改 hugegraph.properties
backend=scylladb
serializer=scylladb
# cassandra backend config
cassandra.host=localhost
cassandra.port=9042
cassandra.username=
cassandra.password=
#cassandra.connect_timeout=5
#cassandra.read_timeout=20
#cassandra.keyspace.strategy=SimpleStrategy
#cassandra.keyspace.replication=3
由于 scylladb 数据库本身就是基于 cassandra 的"优化版",如果用户未安装 scylladb,也可以直接使用 cassandra 作为后端存储,只需要把 backend 和 serializer 修改为 scylladb,host 和 post 指向 cassandra 集群的 seeds 和 port 即可,但是并不建议这样做,这样发挥不出 scylladb 本身的优势了。
初始化数据库(第一次启动时或在 conf/graphs/
下手动添加了新配置时需要进行初始化)
cd *hugegraph-${version}
bin/init-store.sh
启动 server
bin/start-hugegraph.sh
Starting HugeGraphServer...
Connecting to HugeGraphServer (http://127.0.0.1:8080/graphs)....OK
5.1.7 启动 server 的时候创建示例图
在脚本启动时候携带 -p true
参数,表示 preload, 即创建示例图图
bin/start-hugegraph.sh -p true
Starting HugeGraphServer in daemon mode...
Connecting to HugeGraphServer (http://127.0.0.1:8080/graphs)......OK
并且使用 RESTful API 请求 HugeGraphServer
得到如下结果:
> curl "http://localhost:8080/graphs/hugegraph/graph/vertices" | gunzip
{"vertices":[{"id":"2:lop","label":"software","type":"vertex","properties":{"name":"lop","lang":"java","price":328}},{"id":"1:josh","label":"person","type":"vertex","properties":{"name":"josh","age":32,"city":"Beijing"}},{"id":"1:marko","label":"person","type":"vertex","properties":{"name":"marko","age":29,"city":"Beijing"}},{"id":"1:peter","label":"person","type":"vertex","properties":{"name":"peter","age":35,"city":"Shanghai"}},{"id":"1:vadas","label":"person","type":"vertex","properties":{"name":"vadas","age":27,"city":"Hongkong"}},{"id":"2:ripple","label":"software","type":"vertex","properties":{"name":"ripple","lang":"java","price":199}}]}
代表创建示例图成功。
5.2 使用 Docker
在 3.3 使用 Docker 容器中,我们已经介绍了如何使用 docker
部署 hugegraph-server
, 我们还可以使用其他的后端存储或者设置参数在 sever 启动的时候加载样例图
5.2.1 使用 Cassandra 作为后端
点击展开/折叠 Cassandra 配置及启动方法
在使用 Docker 的时候,我们可以使用 Cassandra 作为后端存储。我们更加推荐直接使用 docker-compose 来对于 server 以及 Cassandra 进行统一管理
样例的 docker-compose.yml
可以在 github 中获取,使用 docker-compose up -d
启动。(如果使用 cassandra 4.0 版本作为后端存储,则需要大约两个分钟初始化,请耐心等待)
version: "3"
services:
server:
image: hugegraph/hugegraph
container_name: cas-server
ports:
- 8080:8080
environment:
hugegraph.backend: cassandra
hugegraph.serializer: cassandra
hugegraph.cassandra.host: cas-cassandra
hugegraph.cassandra.port: 9042
networks:
- ca-network
depends_on:
- cassandra
healthcheck:
test: ["CMD", "bin/gremlin-console.sh", "--" ,"-e", "scripts/remote-connect.groovy"]
interval: 10s
timeout: 30s
retries: 3
cassandra:
image: cassandra:4
container_name: cas-cassandra
ports:
- 7000:7000
- 9042:9042
security_opt:
- seccomp:unconfined
networks:
- ca-network
healthcheck:
test: ["CMD", "cqlsh", "--execute", "describe keyspaces;"]
interval: 10s
timeout: 30s
retries: 5
networks:
ca-network:
volumes:
hugegraph-data:
在这个 yaml 中,需要在环境变量中以 hugegraph.<parameter_name>
的形式进行参数传递,配置 Cassandra 相关的参数。
具体来说,在 hugegraph.properties
配置文件中,提供了 backend=xxx
, cassandra.host=xxx
等配置项,为了配置这些配置项,在传递环境变量的过程之中,我们需要在这些配置项前加上 hugegrpah.
,即 hugegraph.backend
和 hugegraph.cassandra.host
。
其他配置可以参照 4 配置
5.2.2 启动 server 的时候创建示例图
在 docker 启动的时候设置环境变量 PRELOAD=true
, 从而实现启动脚本的时候加载数据。
使用
docker run
使用
docker run -itd --name=server -p 8080:8080 -e PRELOAD=true hugegraph/hugegraph:1.5.0
使用
docker-compose
创建
docker-compose.yml
,具体文件如下,在环境变量中设置 PRELOAD=true。其中,example.groovy
是一个预定义的脚本,用于预加载样例数据。如果有需要,可以通过挂载新的example.groovy
脚本改变预加载的数据。version: '3' services: server: image: hugegraph/hugegraph:1.5.0 container_name: server environment: - PRELOAD=true volumes: - /path/to/yourscript:/hugegraph/scripts/example.groovy ports: - 8080:8080
使用命令
docker-compose up -d
启动容器
使用 RESTful API 请求 HugeGraphServer
得到如下结果:
> curl "http://localhost:8080/graphs/hugegraph/graph/vertices" | gunzip
{"vertices":[{"id":"2:lop","label":"software","type":"vertex","properties":{"name":"lop","lang":"java","price":328}},{"id":"1:josh","label":"person","type":"vertex","properties":{"name":"josh","age":32,"city":"Beijing"}},{"id":"1:marko","label":"person","type":"vertex","properties":{"name":"marko","age":29,"city":"Beijing"}},{"id":"1:peter","label":"person","type":"vertex","properties":{"name":"peter","age":35,"city":"Shanghai"}},{"id":"1:vadas","label":"person","type":"vertex","properties":{"name":"vadas","age":27,"city":"Hongkong"}},{"id":"2:ripple","label":"software","type":"vertex","properties":{"name":"ripple","lang":"java","price":199}}]}
代表创建示例图成功。
6 访问 Server
6.1 服务启动状态校验
jps
查看服务进程
jps
6475 HugeGraphServer
curl
请求 RESTful API
echo `curl -o /dev/null -s -w %{http_code} "http://localhost:8080/graphs/hugegraph/graph/vertices"`
返回结果 200,代表 server 启动正常
6.2 请求 Server
HugeGraphServer 的 RESTful API 包括多种类型的资源,典型的包括 graph、schema、gremlin、traverser 和 task
graph
包含vertices
、edges
schema
包含vertexlabels
、propertykeys
、edgelabels
、indexlabels
gremlin
包含各种Gremlin
语句,如g.v()
,可以同步或者异步执行traverser
包含各种高级查询,包括最短路径、交叉点、N 步可达邻居等task
包含异步任务的查询和删除
6.2.1 获取 hugegraph
的顶点及相关属性
curl http://localhost:8080/graphs/hugegraph/graph/vertices
说明
由于图的点和边很多,对于 list 型的请求,比如获取所有顶点,获取所有边等,Server 会将数据压缩再返回,所以使用 curl 时得到一堆乱码,可以重定向至
gunzip
进行解压。推荐使用 Chrome 浏览器 + Restlet 插件发送 HTTP 请求进行测试。curl "http://localhost:8080/graphs/hugegraph/graph/vertices" | gunzip
当前 HugeGraphServer 的默认配置只能是本机访问,可以修改配置,使其能在其他机器访问。
vim conf/rest-server.properties restserver.url=http://0.0.0.0:8080
响应体如下:
{
"vertices": [
{
"id": "2lop",
"label": "software",
"type": "vertex",
"properties": {
"price": [
{
"id": "price",
"value": 328
}
],
"name": [
{
"id": "name",
"value": "lop"
}
],
"lang": [
{
"id": "lang",
"value": "java"
}
]
}
},
{
"id": "1josh",
"label": "person",
"type": "vertex",
"properties": {
"name": [
{
"id": "name",
"value": "josh"
}
],
"age": [
{
"id": "age",
"value": 32
}
]
}
},
...
]
}
详细的 API 请参考 RESTful-API 文档。
另外也可以通过访问 localhost:8080/swagger-ui/index.html
查看 API。
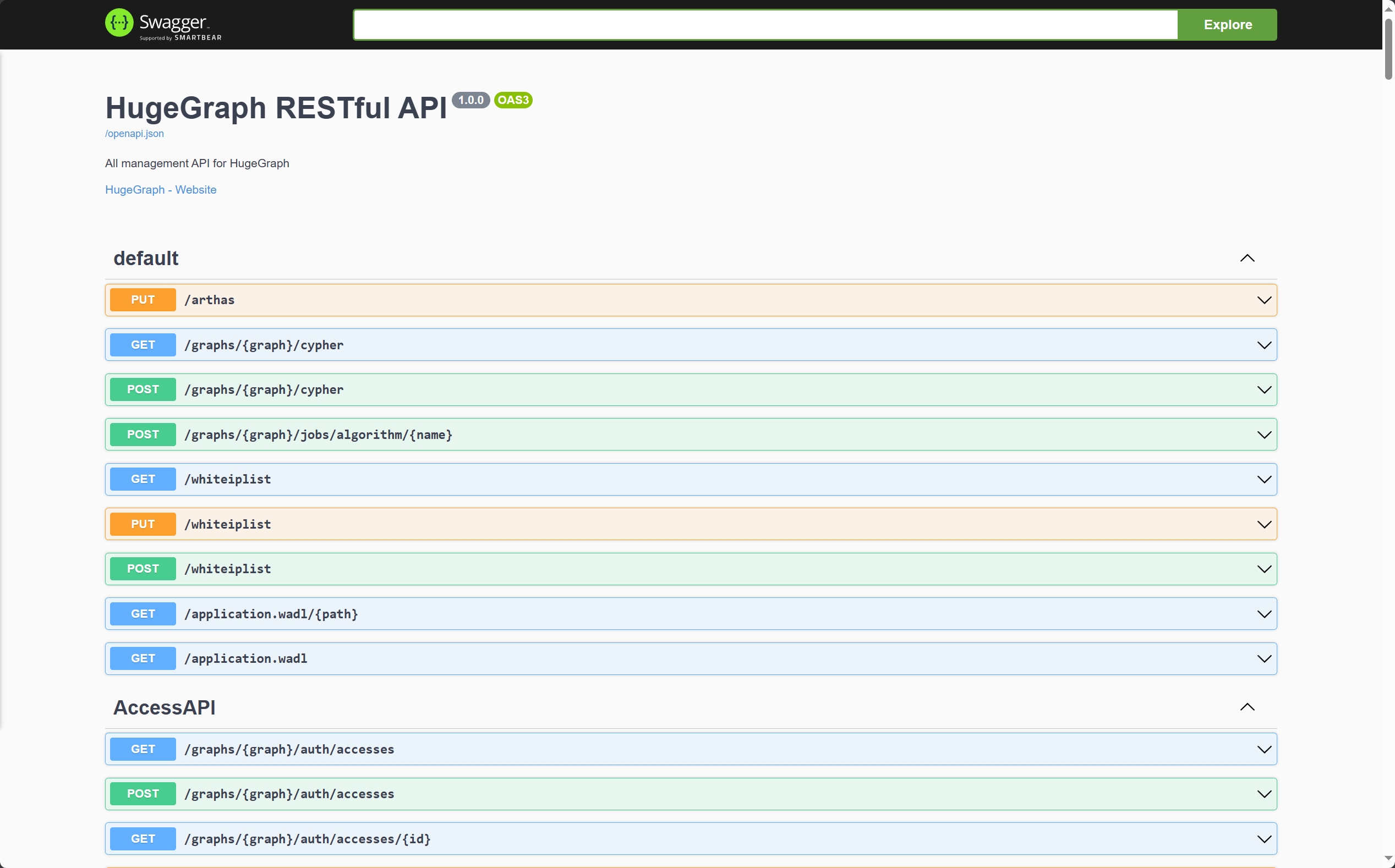
在使用 Swagger UI 调试 HugeGraph 提供的 API 时,如果 HugeGraph Server 开启了鉴权模式,可以在 Swagger 页面输入鉴权信息。
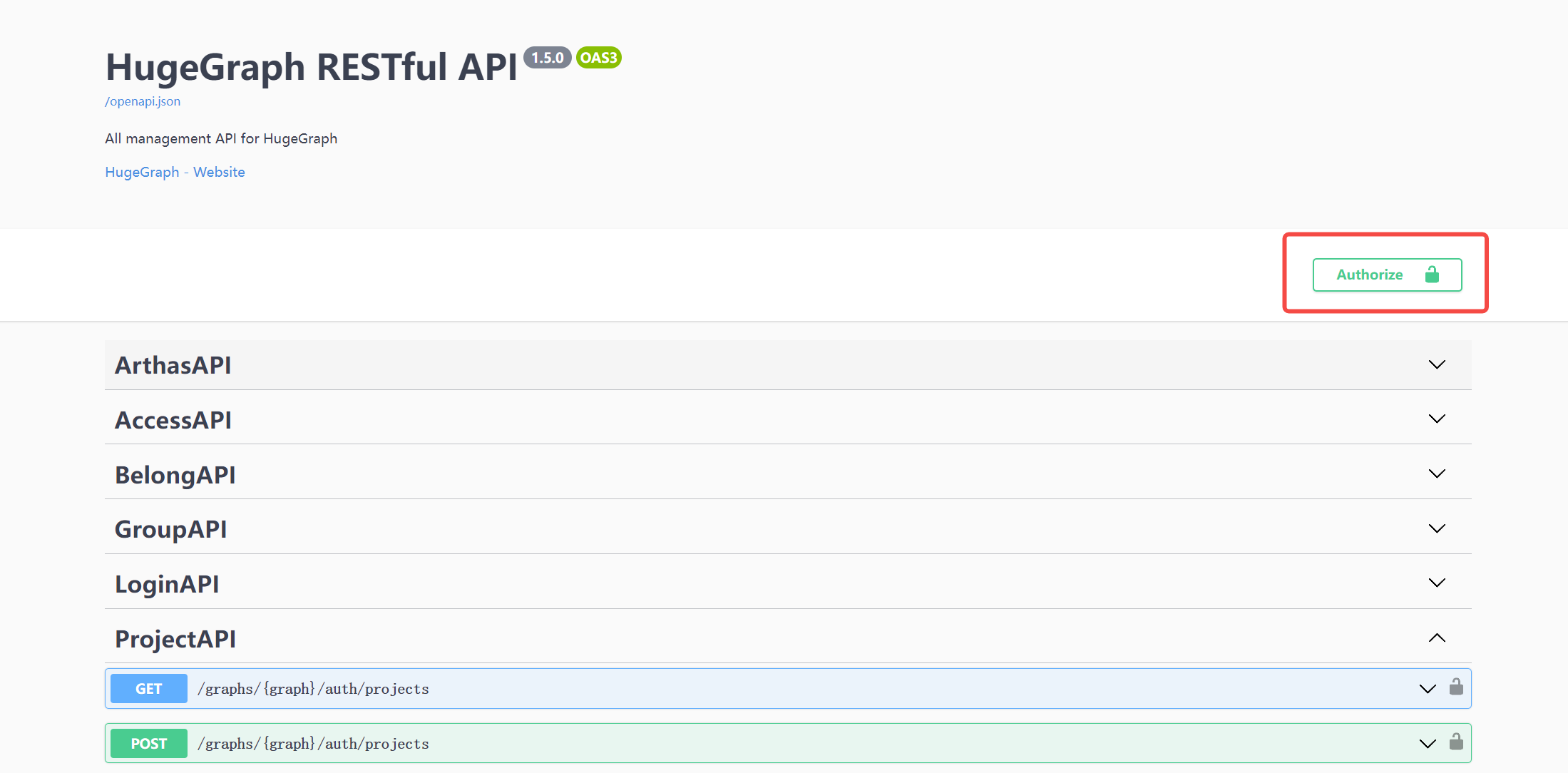
当前 HugeGraph 支持基于 Basic 和 Bearer 两种形式设置鉴权信息。
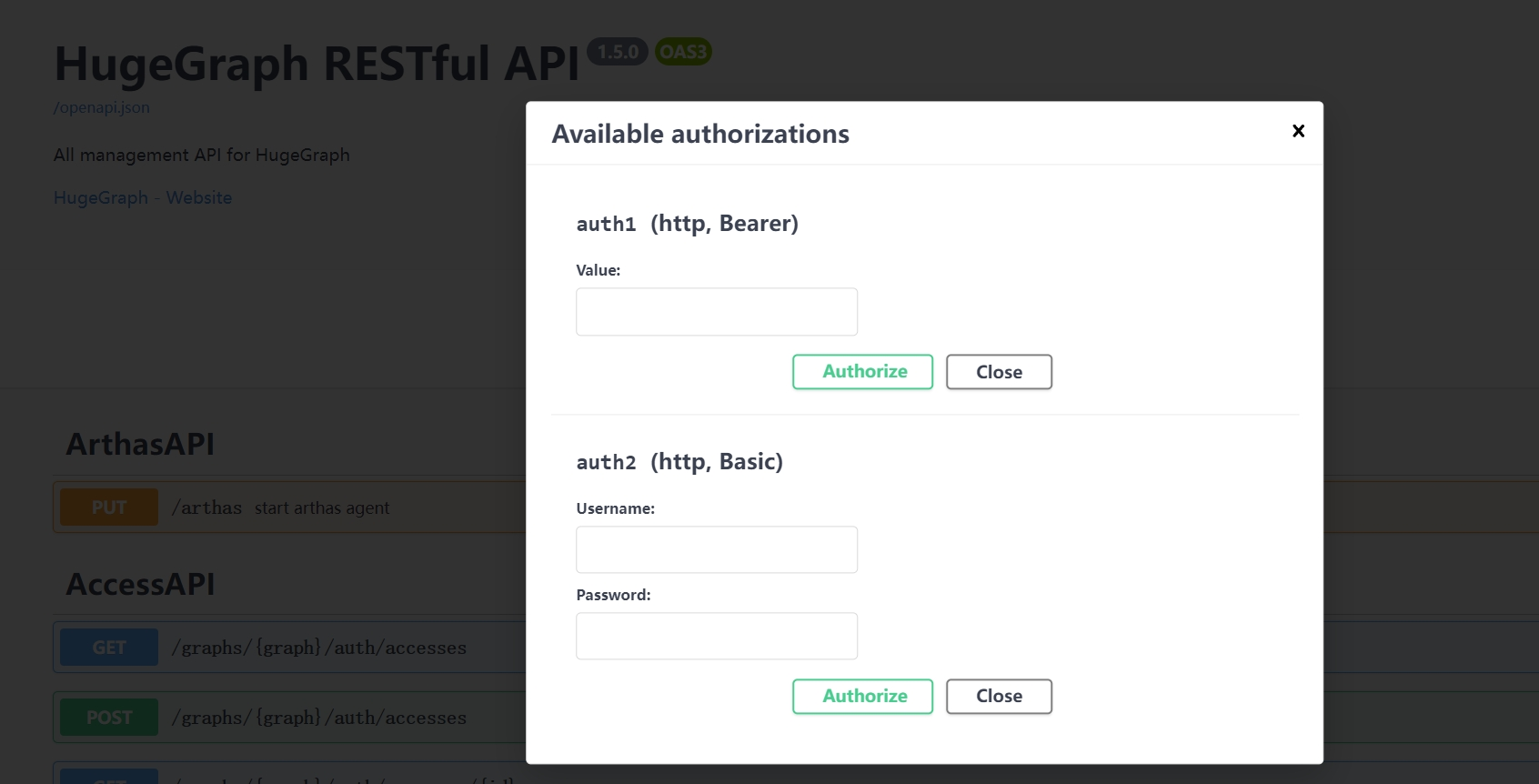
7 停止 Server
$cd *hugegraph-${version}
$bin/stop-hugegraph.sh
8 使用 IntelliJ IDEA 调试 Server
2 - HugeGraph-Loader Quick Start
1 HugeGraph-Loader 概述
HugeGraph-Loader 是 HugeGraph 的数据导入组件,能够将多种数据源的数据转化为图的顶点和边并批量导入到图数据库中。
目前支持的数据源包括:
- 本地磁盘文件或目录,支持 TEXT、CSV 和 JSON 格式的文件,支持压缩文件
- HDFS 文件或目录,支持压缩文件
- 主流关系型数据库,如 MySQL、PostgreSQL、Oracle、SQL Server
本地磁盘文件和 HDFS 文件支持断点续传。
后面会具体说明。
注意:使用 HugeGraph-Loader 需要依赖 HugeGraph Server 服务,下载和启动 Server 请参考 HugeGraph-Server Quick Start
2 获取 HugeGraph-Loader
有两种方式可以获取 HugeGraph-Loader:
- 使用 Docker 镜像 (便于测试)
- 下载已编译的压缩包
- 克隆源码编译安装
2.1 使用 Docker 镜像 (便于测试)
我们可以使用 docker run -itd --name loader hugegraph/loader:1.5.0
部署 loader 服务。对于需要加载的数据,则可以通过挂载 -v /path/to/data/file:/loader/file
或者 docker cp
的方式将文件复制到 loader 容器内部。
或者使用 docker-compose 启动 loader, 启动命令为 docker-compose up -d
, 样例的 docker-compose.yml 如下所示:
version: '3'
services:
server:
image: hugegraph/hugegraph:1.5.0
container_name: server
ports:
- 8080:8080
hubble:
image: hugegraph/hubble:1.5.0
container_name: hubble
ports:
- 8088:8088
loader:
image: hugegraph/loader:1.5.0
container_name: loader
# mount your own data here
# volumes:
# - /path/to/data/file:/loader/file
具体的数据导入流程可以参考 4.5 使用 docker 导入
注意:
hugegraph-loader 的 docker 镜像是一个便捷版本,用于快速启动 loader,并不是官方发布物料包方式。你可以从 ASF Release Distribution Policy 中得到更多细节。
推荐使用
release tag
(如1.5.0
) 以获取稳定版。使用latest
tag 可以使用开发中的最新功能。
2.2 下载已编译的压缩包
下载最新版本的 HugeGraph-Toolchain
Release 包,里面包含了 loader + tool + hubble
全套工具,如果你已经下载,可跳过重复步骤
wget https://downloads.apache.org/incubator/hugegraph/{version}/apache-hugegraph-toolchain-incubating-{version}.tar.gz
tar zxf *hugegraph*.tar.gz
2.3 克隆源码编译安装
克隆最新版本的 HugeGraph-Loader 源码包:
# 1. get from github
git clone https://github.com/apache/hugegraph-toolchain.git
# 2. get from direct url (please choose the **latest release** version)
wget https://downloads.apache.org/incubator/hugegraph/{version}/apache-hugegraph-toolchain-incubating-{version}-src.tar.gz
点击展开/折叠 手动安装 ojdbc 方法
由于 Oracle ojdbc license 的限制,需要手动安装 ojdbc 到本地 maven 仓库。 访问 Oracle jdbc 下载 页面。选择 Oracle Database 12c Release 2 (12.2.0.1) drivers,如下图所示。
打开链接后,选择“ojdbc8.jar”
把 ojdbc8 安装到本地 maven 仓库,进入ojdbc8.jar
所在目录,执行以下命令。
mvn install:install-file -Dfile=./ojdbc8.jar -DgroupId=com.oracle -DartifactId=ojdbc8 -Dversion=12.2.0.1 -Dpackaging=jar
编译生成 tar 包:
cd hugegraph-loader
mvn clean package -DskipTests
3 使用流程
使用 HugeGraph-Loader 的基本流程分为以下几步:
- 编写图模型
- 准备数据文件
- 编写输入源映射文件
- 执行命令导入
3.1 编写图模型
这一步是建模的过程,用户需要对自己已有的数据和想要创建的图模型有一个清晰的构想,然后编写 schema 建立图模型。
比如想创建一个拥有两类顶点及两类边的图,顶点是"人"和"软件",边是"人认识人"和"人创造软件",并且这些顶点和边都带有一些属性,比如顶点"人"有:“姓名”、“年龄"等属性, “软件"有:“名字”、“售卖价格"等属性;边"认识"有:“日期"属性等。
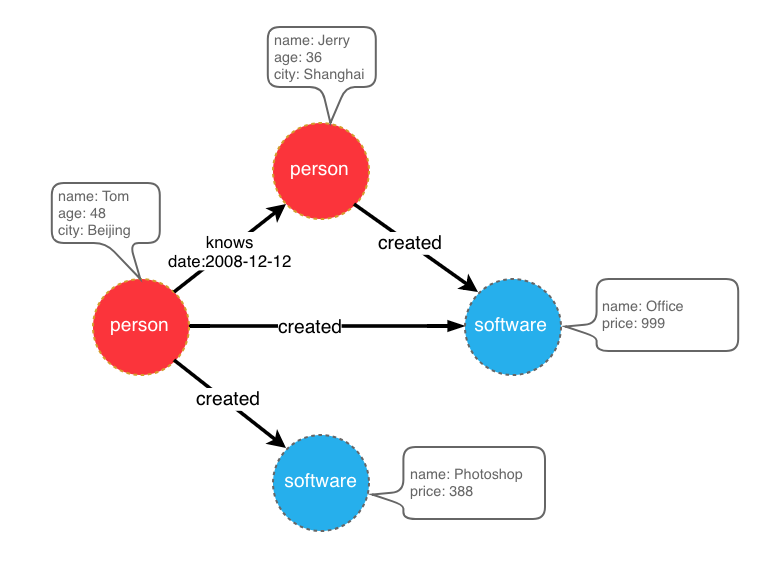
示例图模型
在设计好了图模型之后,我们可以用groovy
编写出schema
的定义,并保存至文件中,这里命名为schema.groovy
。
// 创建一些属性
schema.propertyKey("name").asText().ifNotExist().create();
schema.propertyKey("age").asInt().ifNotExist().create();
schema.propertyKey("city").asText().ifNotExist().create();
schema.propertyKey("date").asText().ifNotExist().create();
schema.propertyKey("price").asDouble().ifNotExist().create();
// 创建 person 顶点类型,其拥有三个属性:name, age, city,主键是 name
schema.vertexLabel("person").properties("name", "age", "city").primaryKeys("name").ifNotExist().create();
// 创建 software 顶点类型,其拥有两个属性:name, price,主键是 name
schema.vertexLabel("software").properties("name", "price").primaryKeys("name").ifNotExist().create();
// 创建 knows 边类型,这类边是从 person 指向 person 的
schema.edgeLabel("knows").sourceLabel("person").targetLabel("person").ifNotExist().create();
// 创建 created 边类型,这类边是从 person 指向 software 的
schema.edgeLabel("created").sourceLabel("person").targetLabel("software").ifNotExist().create();
关于 schema 的详细说明请参考 hugegraph-client 中对应部分。
3.2 准备数据
目前 HugeGraph-Loader 支持的数据源包括:
- 本地磁盘文件或目录
- HDFS 文件或目录
- 部分关系型数据库
- Kafka topic
3.2.1 数据源结构
3.2.1.1 本地磁盘文件或目录
用户可以指定本地磁盘文件作为数据源,如果数据分散在多个文件中,也支持以某个目录作为数据源,但暂时不支持以多个目录作为数据源。
比如:我的数据分散在多个文件中,part-0、part-1 … part-n,要想执行导入,必须保证它们是放在一个目录下的。然后在 loader 的映射文件中,将path
指定为该目录即可。
支持的文件格式包括:
- TEXT
- CSV
- JSON
TEXT 是自定义分隔符的文本文件,第一行通常是标题,记录了每一列的名称,也允许没有标题行(在映射文件中指定)。其余的每行代表一条记录,会被转化为一个顶点/边;行的每一列对应一个字段,会被转化为顶点/边的 id、label 或属性;
示例如下:
id|name|lang|price|ISBN
1|lop|java|328|ISBN978-7-107-18618-5
2|ripple|java|199|ISBN978-7-100-13678-5
CSV 是分隔符为逗号,
的 TEXT 文件,当列值本身包含逗号时,该列值需要用双引号包起来,如:
marko,29,Beijing
"li,nary",26,"Wu,han"
JSON 文件要求每一行都是一个 JSON 串,且每行的格式需保持一致。
{"source_name": "marko", "target_name": "vadas", "date": "20160110", "weight": 0.5}
{"source_name": "marko", "target_name": "josh", "date": "20130220", "weight": 1.0}
3.2.1.2 HDFS 文件或目录
用户也可以指定 HDFS 文件或目录作为数据源,上面关于本地磁盘文件或目录
的要求全部适用于这里。除此之外,鉴于 HDFS 上通常存储的都是压缩文件,loader 也提供了对压缩文件的支持,并且本地磁盘文件或目录
同样支持压缩文件。
目前支持的压缩文件类型包括:GZIP、BZ2、XZ、LZMA、SNAPPY_RAW、SNAPPY_FRAMED、Z、DEFLATE、LZ4_BLOCK、LZ4_FRAMED、ORC 和 PARQUET。
3.2.1.3 主流关系型数据库
loader 还支持以部分关系型数据库作为数据源,目前支持 MySQL、PostgreSQL、Oracle 和 SQL Server。
但目前对表结构要求较为严格,如果导入过程中需要做关联查询,这样的表结构是不允许的。关联查询的意思是:在读到表的某行后,发现某列的值不能直接使用(比如外键),需要再去做一次查询才能确定该列的真实值。
举个例子:假设有三张表,person、software 和 created
// person 表结构
id | name | age | city
// software 表结构
id | name | lang | price
// created 表结构
id | p_id | s_id | date
如果在建模(schema)时指定 person 或 software 的 id 策略是 PRIMARY_KEY,选择以 name 作为 primary keys(注意:这是 hugegraph 中 vertexlabel 的概念),在导入边数据时,由于需要拼接出源顶点和目标顶点的 id,必须拿着 p_id/s_id 去 person/software 表中查到对应的 name,这种需要做额外查询的表结构的情况,loader 暂时是不支持的。这时可以采用以下两种方式替代:
- 仍然指定 person 和 software 的 id 策略为 PRIMARY_KEY,但是以 person 表和 software 表的 id 列作为顶点的主键属性,这样导入边时直接使用 p_id 和 s_id 和顶点的 label 拼接就能生成 id 了;
- 指定 person 和 software 的 id 策略为 CUSTOMIZE,然后直接以 person 表和 software 表的 id 列作为顶点 id,这样导入边时直接使用 p_id 和 s_id 即可;
关键点就是要让边能直接使用 p_id 和 s_id,不要再去查一次。
3.2.2 准备顶点和边数据
3.2.2.1 顶点数据
顶点数据文件由一行一行的数据组成,一般每一行作为一个顶点,每一列会作为顶点属性。下面以 CSV 格式作为示例进行说明。
- person 顶点数据(数据本身不包含 header)
Tom,48,Beijing
Jerry,36,Shanghai
- software 顶点数据(数据本身包含 header)
name,price
Photoshop,999
Office,388
3.2.2.2 边数据
边数据文件由一行一行的数据组成,一般每一行作为一条边,其中有部分列会作为源顶点和目标顶点的 id,其他列作为边属性。下面以 JSON 格式作为示例进行说明。
- knows 边数据
{"source_name": "Tom", "target_name": "Jerry", "date": "2008-12-12"}
- created 边数据
{"source_name": "Tom", "target_name": "Photoshop"}
{"source_name": "Tom", "target_name": "Office"}
{"source_name": "Jerry", "target_name": "Office"}
3.3 编写数据源映射文件
3.3.1 映射文件概述
输入源的映射文件用于描述如何将输入源数据与图的顶点类型/边类型建立映射关系,以JSON
格式组织,由多个映射块组成,其中每一个映射块都负责将一个输入源映射为顶点和边。
具体而言,每个映射块包含一个输入源和多个顶点映射与边映射块,输入源块对应上面介绍的本地磁盘文件或目录
、HDFS 文件或目录
和关系型数据库
,负责描述数据源的基本信息,比如数据在哪,是什么格式的,分隔符是什么等。顶点映射/边映射与该输入源绑定,可以选择输入源的哪些列,哪些列作为 id、哪些列作为属性,以及每一列映射成什么属性,列的值映射成属性的什么值等等。
以最通俗的话讲,每一个映射块描述了:要导入的文件在哪,文件的每一行要作为哪一类顶点/边,文件的哪些列是需要导入的,以及这些列对应顶点/边的什么属性等。
注意:0.11.0 版本以前的映射文件与 0.11.0 以后的格式变化较大,为表述方便,下面称 0.11.0 以前的映射文件(格式)为 1.0 版本,0.11.0 以后的为 2.0 版本。并且若无特殊说明,“映射文件”表示的是 2.0 版本的。
点击展开/折叠 2.0 版本的映射文件的框架
{
"version": "2.0",
"structs": [
{
"id": "1",
"input": {
},
"vertices": [
{},
{}
],
"edges": [
{},
{}
]
}
]
}
这里直接给出两个版本的映射文件(描述了上面图模型和数据文件)
点击展开/折叠 2.0 版本的映射文件
{
"version": "2.0",
"structs": [
{
"id": "1",
"skip": false,
"input": {
"type": "FILE",
"path": "vertex_person.csv",
"file_filter": {
"extensions": [
"*"
]
},
"format": "CSV",
"delimiter": ",",
"date_format": "yyyy-MM-dd HH:mm:ss",
"time_zone": "GMT+8",
"skipped_line": {
"regex": "(^#|^//).*|"
},
"compression": "NONE",
"header": [
"name",
"age",
"city"
],
"charset": "UTF-8",
"list_format": {
"start_symbol": "[",
"elem_delimiter": "|",
"end_symbol": "]"
}
},
"vertices": [
{
"label": "person",
"skip": false,
"id": null,
"unfold": false,
"field_mapping": {},
"value_mapping": {},
"selected": [],
"ignored": [],
"null_values": [
""
],
"update_strategies": {}
}
],
"edges": []
},
{
"id": "2",
"skip": false,
"input": {
"type": "FILE",
"path": "vertex_software.csv",
"file_filter": {
"extensions": [
"*"
]
},
"format": "CSV",
"delimiter": ",",
"date_format": "yyyy-MM-dd HH:mm:ss",
"time_zone": "GMT+8",
"skipped_line": {
"regex": "(^#|^//).*|"
},
"compression": "NONE",
"header": null,
"charset": "UTF-8",
"list_format": {
"start_symbol": "",
"elem_delimiter": ",",
"end_symbol": ""
}
},
"vertices": [
{
"label": "software",
"skip": false,
"id": null,
"unfold": false,
"field_mapping": {},
"value_mapping": {},
"selected": [],
"ignored": [],
"null_values": [
""
],
"update_strategies": {}
}
],
"edges": []
},
{
"id": "3",
"skip": false,
"input": {
"type": "FILE",
"path": "edge_knows.json",
"file_filter": {
"extensions": [
"*"
]
},
"format": "JSON",
"delimiter": null,
"date_format": "yyyy-MM-dd HH:mm:ss",
"time_zone": "GMT+8",
"skipped_line": {
"regex": "(^#|^//).*|"
},
"compression": "NONE",
"header": null,
"charset": "UTF-8",
"list_format": null
},
"vertices": [],
"edges": [
{
"label": "knows",
"skip": false,
"source": [
"source_name"
],
"unfold_source": false,
"target": [
"target_name"
],
"unfold_target": false,
"field_mapping": {
"source_name": "name",
"target_name": "name"
},
"value_mapping": {},
"selected": [],
"ignored": [],
"null_values": [
""
],
"update_strategies": {}
}
]
},
{
"id": "4",
"skip": false,
"input": {
"type": "FILE",
"path": "edge_created.json",
"file_filter": {
"extensions": [
"*"
]
},
"format": "JSON",
"delimiter": null,
"date_format": "yyyy-MM-dd HH:mm:ss",
"time_zone": "GMT+8",
"skipped_line": {
"regex": "(^#|^//).*|"
},
"compression": "NONE",
"header": null,
"charset": "UTF-8",
"list_format": null
},
"vertices": [],
"edges": [
{
"label": "created",
"skip": false,
"source": [
"source_name"
],
"unfold_source": false,
"target": [
"target_name"
],
"unfold_target": false,
"field_mapping": {
"source_name": "name",
"target_name": "name"
},
"value_mapping": {},
"selected": [],
"ignored": [],
"null_values": [
""
],
"update_strategies": {}
}
]
}
]
}
点击展开/折叠 1.0 版本的映射文件
{
"vertices": [
{
"label": "person",
"input": {
"type": "file",
"path": "vertex_person.csv",
"format": "CSV",
"header": ["name", "age", "city"],
"charset": "UTF-8"
}
},
{
"label": "software",
"input": {
"type": "file",
"path": "vertex_software.csv",
"format": "CSV"
}
}
],
"edges": [
{
"label": "knows",
"source": ["source_name"],
"target": ["target_name"],
"input": {
"type": "file",
"path": "edge_knows.json",
"format": "JSON"
},
"field_mapping": {
"source_name": "name",
"target_name": "name"
}
},
{
"label": "created",
"source": ["source_name"],
"target": ["target_name"],
"input": {
"type": "file",
"path": "edge_created.json",
"format": "JSON"
},
"field_mapping": {
"source_name": "name",
"target_name": "name"
}
}
]
}
映射文件 1.0 版本是以顶点和边为中心,设置输入源;而 2.0 版本是以输入源为中心,设置顶点和边映射。有些输入源(比如一个文件)既能生成顶点,也能生成边,如果用 1.0 版的格式写,就需要在 vertex 和 edge 映射块中各写一次 input 块,这两次的 input 块是完全一样的;而 2.0 版本只需要写一次 input。所以 2.0 版相比于 1.0 版,能省掉一些 input 的重复书写。
在 hugegraph-loader-{version} 的 bin 目录下,有一个脚本工具 mapping-convert.sh
能直接将 1.0 版本的映射文件转换为 2.0 版本的,使用方式如下:
bin/mapping-convert.sh struct.json
会在 struct.json 的同级目录下生成一个 struct-v2.json。
3.3.2 输入源
输入源目前分为四类:FILE、HDFS、JDBC、KAFKA,由type
节点区分,我们称为本地文件输入源、HDFS 输入源、JDBC 输入源和 KAFKA 输入源,下面分别介绍。
3.3.2.1 本地文件输入源
- id: 输入源的 id,该字段用于支持一些内部功能,非必填(未填时会自动生成),强烈建议写上,对于调试大有裨益;
- skip: 是否跳过该输入源,由于 JSON 文件无法添加注释,如果某次导入时不想导入某个输入源,但又不想删除该输入源的配置,则可以设置为 true 将其跳过,默认为 false,非必填;
- input: 输入源映射块,复合结构
- type: 输入源类型,必须填 file 或 FILE;
- path: 本地文件或目录的路径,绝对路径或相对于映射文件的相对路径,建议使用绝对路径,必填;
- file_filter: 从
path
中筛选复合条件的文件,复合结构,目前只支持配置扩展名,用子节点extensions
表示,默认为”*",表示保留所有文件; - format: 本地文件的格式,可选值为 CSV、TEXT 及 JSON,必须大写,必填;
- header: 文件各列的列名,如不指定则会以数据文件第一行作为 header;当文件本身有标题且又指定了 header,文件的第一行会被当作普通的数据行;JSON 文件不需要指定 header,选填;
- delimiter: 文件行的列分隔符,默认以逗号
","
作为分隔符,JSON
文件不需要指定,选填; - charset: 文件的编码字符集,默认
UTF-8
,选填; - date_format: 自定义的日期格式,默认值为 yyyy-MM-dd HH:mm:ss,选填;如果日期是以时间戳的形式呈现的,此项须写为
timestamp
(固定写法); - time_zone: 设置日期数据是处于哪个时区的,默认值为
GMT+8
,选填; - skipped_line: 想跳过的行,复合结构,目前只能配置要跳过的行的正则表达式,用子节点
regex
描述,默认不跳过任何行,选填; - compression: 文件的压缩格式,可选值为 NONE、GZIP、BZ2、XZ、LZMA、SNAPPY_RAW、SNAPPY_FRAMED、Z、DEFLATE、LZ4_BLOCK、LZ4_FRAMED、ORC 和 PARQUET,默认为 NONE,表示非压缩文件,选填;
- list_format: 当文件 (非 JSON ) 的某列是集合结构时(对应图中的 PropertyKey 的 Cardinality 为 Set 或 List),可以用此项设置该列的起始符、分隔符、结束符,复合结构:
- start_symbol: 集合结构列的起始符 (默认值是
[
, JSON 格式目前不支持指定) - elem_delimiter: 集合结构列的分隔符 (默认值是
|
, JSON 格式目前只支持原生,
分隔) - end_symbol: 集合结构列的结束符 (默认值是
]
, JSON 格式目前不支持指定)
- start_symbol: 集合结构列的起始符 (默认值是
3.3.2.2 HDFS 输入源
上述本地文件输入源
的节点及含义这里基本都适用,下面仅列出 HDFS 输入源不一样的和特有的节点。
- type: 输入源类型,必须填 hdfs 或 HDFS,必填;
- path: HDFS 文件或目录的路径,必须是 HDFS 的绝对路径,必填;
- core_site_path: HDFS 集群的 core-site.xml 文件路径,重点要指明 NameNode 的地址(
fs.default.name
),以及文件系统的实现(fs.hdfs.impl
);
3.3.2.3 JDBC 输入源
前面说到过支持多种关系型数据库,但由于它们的映射结构非常相似,故统称为 JDBC 输入源,然后用vendor
节点区分不同的数据库。
- type: 输入源类型,必须填 jdbc 或 JDBC,必填;
- vendor: 数据库类型,可选项为 [MySQL、PostgreSQL、Oracle、SQLServer],不区分大小写,必填;
- driver: jdbc 使用的 driver 类型,必填;
- url: jdbc 要连接的数据库的 url,必填;
- database: 要连接的数据库名,必填;
- schema: 要连接的 schema 名,不同的数据库要求不一样,下面详细说明;
- table: 要连接的表名,
custom_sql
和table
参数必须填其中一个; - custom_sql: 自定义 SQL 语句,
custom_sql
和table
参数必须填其中一个; - username: 连接数据库的用户名,必填;
- password: 连接数据库的密码,必填;
- batch_size: 按页获取表数据时的一页的大小,默认为 500,选填;
MYSQL
节点 | 固定值或常见值 |
---|---|
vendor | MYSQL |
driver | com.mysql.cj.jdbc.Driver |
url | jdbc:mysql://127.0.0.1:3306 |
schema: 可空,若填写必须与 database 的值一样
POSTGRESQL
节点 | 固定值或常见值 |
---|---|
vendor | POSTGRESQL |
driver | org.postgresql.Driver |
url | jdbc:postgresql://127.0.0.1:5432 |
schema: 可空,默认值为“public”
ORACLE
节点 | 固定值或常见值 |
---|---|
vendor | ORACLE |
driver | oracle.jdbc.driver.OracleDriver |
url | jdbc:oracle:thin:@127.0.0.1:1521 |
schema: 可空,默认值与用户名相同
SQLSERVER
节点 | 固定值或常见值 |
---|---|
vendor | SQLSERVER |
driver | com.microsoft.sqlserver.jdbc.SQLServerDriver |
url | jdbc:sqlserver://127.0.0.1:1433 |
schema: 必填
3.3.2.4 Kafka 输入源
- type:输入源类型,必须填
kafka
或KAFKA
,必填; - bootstrap_server:设置 kafka bootstrap server 列表;
- topic:订阅的 topic;
- group:Kafka 消费者组;
- from_beginning:设置是否从头开始读取;
- format:本地文件的格式,可选值为 CSV、TEXT 及 JSON,必须大写,必填;
- header:文件各列的列名,如不指定则会以数据文件第一行作为 header;当文件本身有标题且又指定了 header,文件的第一行会被当作普通的数据行;JSON 文件不需要指定 header,选填;
- delimiter:文件行的列分隔符,默认以逗号”,“作为分隔符,JSON 文件不需要指定,选填;
- charset:文件的编码字符集,默认 UTF-8,选填;
- date_format:自定义的日期格式,默认值为 yyyy-MM-dd HH:mm:ss,选填;如果日期是以时间戳的形式呈现的,此项须写为 timestamp(固定写法);
- extra_date_formats:自定义的其他日期格式列表,默认为空,选填;列表中每一项都是一个 date_format 指定日期格式的备用日期格式;
- time_zone:置日期数据是处于哪个时区的,默认值为 GMT+8,选填;
- skipped_line:想跳过的行,复合结构,目前只能配置要跳过的行的正则表达式,用子节点 regex 描述,默认不跳过任何行,选填;
- early_stop:某次从 Kafka broker 拉取的记录为空,停止任务,默认为 false,仅用于调试,选填;
3.3.3 顶点和边映射
顶点和边映射的节点(JSON 文件中的一个 key)有很多相同的部分,下面先介绍相同部分,再分别介绍顶点映射
和边映射
的特有节点。
相同部分的节点
- label: 待导入的顶点/边数据所属的
label
,必填; - field_mapping: 将输入源列的列名映射为顶点/边的属性名,选填;
- value_mapping: 将输入源的数据值映射为顶点/边的属性值,选填;
- selected: 选择某些列插入,其他未选中的不插入,不能与
ignored
同时存在,选填; - ignored: 忽略某些列,使其不参与插入,不能与
selected
同时存在,选填; - null_values: 可以指定一些字符串代表空值,比如"NULL”,如果该列对应的顶点/边属性又是一个可空属性,那在构造顶点/边时不会设置该属性的值,选填;
- update_strategies: 如果数据需要按特定方式批量更新时可以对每个属性指定具体的更新策略 (具体见下),选填;
- unfold: 是否将列展开,展开的每一列都会与其他列一起组成一行,相当于是展开成了多行;比如文件的某一列(id 列)的值是
[1,2,3]
,其他列的值是18,Beijing
,当设置了 unfold 之后,这一行就会变成 3 行,分别是:1,18,Beijing
,2,18,Beijing
和3,18,Beijing
。需要注意的是此项只会展开被选作为 id 的列。默认 false,选填;
更新策略支持 8 种 : (需要全大写)
- 数值累加 :
SUM
- 两个数字/日期取更大的:
BIGGER
- 两个数字/日期取更小:
SMALLER
- Set属性取并集:
UNION
- Set属性取交集:
INTERSECTION
- List属性追加元素:
APPEND
- List/Set属性删除元素:
ELIMINATE
- 覆盖已有属性:
OVERRIDE
注意: 如果新导入的属性值为空,会采用已有的旧数据而不会采用空值,效果可以参考如下示例
// JSON 文件中以如下方式指定更新策略
{
"vertices": [
{
"label": "person",
"update_strategies": {
"age": "SMALLER",
"set": "UNION"
},
"input": {
"type": "file",
"path": "vertex_person.txt",
"format": "TEXT",
"header": ["name", "age", "set"]
}
}
]
}
// 1.写入一行带 OVERRIDE 更新策略的数据 (这里 null 代表空)
'a b null null'
// 2.再写一行
'null null c d'
// 3.最后可以得到
'a b c d'
// 如果没有更新策略,则会得到
'null null c d'
注意 : 采用了批量更新的策略后, 磁盘读请求数会大幅上升, 导入速度相比纯写覆盖会慢数倍 (此时HDD磁盘IOPS会成为瓶颈, 建议采用SSD以保证速度)
顶点映射的特有节点
- id: 指定某一列作为顶点的 id 列,当顶点 id 策略为
CUSTOMIZE
时,必填;当 id 策略为PRIMARY_KEY
时,必须为空;
边映射的特有节点
- source: 选择输入源某几列作为源顶点的 id 列,当源顶点的 id 策略为
CUSTOMIZE
时,必须指定某一列作为顶点的 id 列;当源顶点的 id 策略为PRIMARY_KEY
时,必须指定一列或多列用于拼接生成顶点的 id,也就是说,不管是哪种 id 策略,此项必填; - target: 指定某几列作为目标顶点的 id 列,与 source 类似,不再赘述;
- unfold_source: 是否展开文件的 source 列,效果与顶点映射中的类似,不再赘述;
- unfold_target: 是否展开文件的 target 列,效果与顶点映射中的类似,不再赘述;
3.4 执行命令导入
准备好图模型、数据文件以及输入源映射关系文件后,接下来就可以将数据文件导入到图数据库中。
导入过程由用户提交的命令控制,用户可以通过不同的参数控制执行的具体流程。
3.4.1 参数说明
参数 | 默认值 | 是否必传 | 描述信息 |
---|---|---|---|
-f 或 --file | Y | 配置脚本的路径 | |
-g 或 --graph | Y | 图数据库空间 | |
-s 或 --schema | Y | schema 文件路径 | |
-h 或 --host | localhost | HugeGraphServer 的地址 | |
-p 或 --port | 8080 | HugeGraphServer 的端口号 | |
--username | null | 当 HugeGraphServer 开启了权限认证时,当前图的 username | |
--token | null | 当 HugeGraphServer 开启了权限认证时,当前图的 token | |
--protocol | http | 向服务端发请求的协议,可选 http 或 https | |
--trust-store-file | 请求协议为 https 时,客户端的证书文件路径 | ||
--trust-store-password | 请求协议为 https 时,客户端证书密码 | ||
--clear-all-data | false | 导入数据前是否清除服务端的原有数据 | |
--clear-timeout | 240 | 导入数据前清除服务端的原有数据的超时时间 | |
--incremental-mode | false | 是否使用断点续导模式,仅输入源为 FILE 和 HDFS 支持该模式,启用该模式能从上一次导入停止的地方开始导 | |
--failure-mode | false | 失败模式为 true 时,会导入之前失败了的数据,一般来说失败数据文件需要在人工更正编辑好后,再次进行导入 | |
--batch-insert-threads | CPUs | 批量插入线程池大小 (CPUs 是当前 OS 可用可用逻辑核个数) | |
--single-insert-threads | 8 | 单条插入线程池的大小 | |
--max-conn | 4 * CPUs | HugeClient 与 HugeGraphServer 的最大 HTTP 连接数,调整线程的时候建议同时调整此项 | |
--max-conn-per-route | 2 * CPUs | HugeClient 与 HugeGraphServer 每个路由的最大 HTTP 连接数,调整线程的时候建议同时调整此项 | |
--batch-size | 500 | 导入数据时每个批次包含的数据条数 | |
--max-parse-errors | 1 | 最多允许多少行数据解析错误,达到该值则程序退出 | |
--max-insert-errors | 500 | 最多允许多少行数据插入错误,达到该值则程序退出 | |
--timeout | 60 | 插入结果返回的超时时间(秒) | |
--shutdown-timeout | 10 | 多线程停止的等待时间(秒) | |
--retry-times | 0 | 发生特定异常时的重试次数 | |
--retry-interval | 10 | 重试之前的间隔时间(秒) | |
--check-vertex | false | 插入边时是否检查边所连接的顶点是否存在 | |
--print-progress | true | 是否在控制台实时打印导入条数 | |
--dry-run | false | 打开该模式,只解析不导入,通常用于测试 | |
--help | false | 打印帮助信息 |
3.4.2 断点续导模式
通常情况下,Loader 任务都需要较长时间执行,如果因为某些原因导致导入中断进程退出,而下次希望能从中断的点继续导,这就是使用断点续导的场景。
用户设置命令行参数 –incremental-mode 为 true 即打开了断点续导模式。断点续导的关键在于进度文件,导入进程退出的时候,会把退出时刻的导入进度
记录到进度文件中,进度文件位于 ${struct}
目录下,文件名形如 load-progress ${date}
,${struct} 为映射文件的前缀,${date} 为导入开始
的时刻。比如:在 2019-10-10 12:30:30
开始的一次导入任务,使用的映射文件为 struct-example.json
,则进度文件的路径为与 struct-example.json
同级的 struct-example/load-progress 2019-10-10 12:30:30
。
注意:进度文件的生成与 –incremental-mode 是否打开无关,每次导入结束都会生成一个进度文件。
如果数据文件格式都是合法的,是用户自己停止(CTRL + C 或 kill,kill -9 不支持)的导入任务,也就是说没有错误记录的情况下,下一次导入只需要设置 为断点续导即可。
但如果是因为太多数据不合法或者网络异常,达到了 –max-parse-errors 或 –max-insert-errors 的限制,Loader 会把这些插入失败的原始行记录到 失败文件中,用户对失败文件中的数据行修改后,设置 –reload-failure 为 true 即可把这些"失败文件"也当作输入源进行导入(不影响正常的文件的导入), 当然如果修改后的数据行仍然有问题,则会被再次记录到失败文件中(不用担心会有重复行)。
每个顶点映射或边映射有数据插入失败时都会产生自己的失败文件,失败文件又分为解析失败文件(后缀 .parse-error)和插入失败文件(后缀 .insert-error),
它们被保存在 ${struct}/current
目录下。比如映射文件中有一个顶点映射 person 和边映射 knows,它们各有一些错误行,当 Loader 退出后,在
${struct}/current
目录下会看到如下文件:
- person-b4cd32ab.parse-error: 顶点映射 person 解析错误的数据
- person-b4cd32ab.insert-error: 顶点映射 person 插入错误的数据
- knows-eb6b2bac.parse-error: 边映射 knows 解析错误的数据
- knows-eb6b2bac.insert-error: 边映射 knows 插入错误的数据
.parse-error 和 .insert-error 并不总是一起存在的,只有存在解析出错的行才会有 .parse-error 文件,只有存在插入出错的行才会有 .insert-error 文件。
3.4.3 logs 目录文件说明
程序执行过程中各日志及错误数据会写入 hugegraph-loader.log 文件中。
3.4.4 执行命令
运行 bin/hugegraph-loader 并传入参数
bin/hugegraph-loader -g {GRAPH_NAME} -f ${INPUT_DESC_FILE} -s ${SCHEMA_FILE} -h {HOST} -p {PORT}
4 完整示例
下面给出的是 hugegraph-loader 包中 example 目录下的例子。(GitHub 地址)
4.1 准备数据
顶点文件:example/file/vertex_person.csv
marko,29,Beijing
vadas,27,Hongkong
josh,32,Beijing
peter,35,Shanghai
"li,nary",26,"Wu,han"
tom,null,NULL
顶点文件:example/file/vertex_software.txt
id|name|lang|price|ISBN
1|lop|java|328|ISBN978-7-107-18618-5
2|ripple|java|199|ISBN978-7-100-13678-5
边文件:example/file/edge_knows.json
{"source_name": "marko", "target_name": "vadas", "date": "20160110", "weight": 0.5}
{"source_name": "marko", "target_name": "josh", "date": "20130220", "weight": 1.0}
边文件:example/file/edge_created.json
{"aname": "marko", "bname": "lop", "date": "20171210", "weight": 0.4}
{"aname": "josh", "bname": "lop", "date": "20091111", "weight": 0.4}
{"aname": "josh", "bname": "ripple", "date": "20171210", "weight": 1.0}
{"aname": "peter", "bname": "lop", "date": "20170324", "weight": 0.2}
4.2 编写 schema
点击展开/折叠 schema 文件:example/file/schema.groovy
schema.propertyKey("name").asText().ifNotExist().create();
schema.propertyKey("age").asInt().ifNotExist().create();
schema.propertyKey("city").asText().ifNotExist().create();
schema.propertyKey("weight").asDouble().ifNotExist().create();
schema.propertyKey("lang").asText().ifNotExist().create();
schema.propertyKey("date").asText().ifNotExist().create();
schema.propertyKey("price").asDouble().ifNotExist().create();
schema.vertexLabel("person").properties("name", "age", "city").primaryKeys("name").ifNotExist().create();
schema.vertexLabel("software").properties("name", "lang", "price").primaryKeys("name").ifNotExist().create();
schema.indexLabel("personByAge").onV("person").by("age").range().ifNotExist().create();
schema.indexLabel("personByCity").onV("person").by("city").secondary().ifNotExist().create();
schema.indexLabel("personByAgeAndCity").onV("person").by("age", "city").secondary().ifNotExist().create();
schema.indexLabel("softwareByPrice").onV("software").by("price").range().ifNotExist().create();
schema.edgeLabel("knows").sourceLabel("person").targetLabel("person").properties("date", "weight").ifNotExist().create();
schema.edgeLabel("created").sourceLabel("person").targetLabel("software").properties("date", "weight").ifNotExist().create();
schema.indexLabel("createdByDate").onE("created").by("date").secondary().ifNotExist().create();
schema.indexLabel("createdByWeight").onE("created").by("weight").range().ifNotExist().create();
schema.indexLabel("knowsByWeight").onE("knows").by("weight").range().ifNotExist().create();
4.3 编写输入源映射文件example/file/struct.json
点击展开/折叠 源映射文件 example/file/struct.json
{
"vertices": [
{
"label": "person",
"input": {
"type": "file",
"path": "example/file/vertex_person.csv",
"format": "CSV",
"header": ["name", "age", "city"],
"charset": "UTF-8",
"skipped_line": {
"regex": "(^#|^//).*"
}
},
"null_values": ["NULL", "null", ""]
},
{
"label": "software",
"input": {
"type": "file",
"path": "example/file/vertex_software.txt",
"format": "TEXT",
"delimiter": "|",
"charset": "GBK"
},
"id": "id",
"ignored": ["ISBN"]
}
],
"edges": [
{
"label": "knows",
"source": ["source_name"],
"target": ["target_name"],
"input": {
"type": "file",
"path": "example/file/edge_knows.json",
"format": "JSON",
"date_format": "yyyyMMdd"
},
"field_mapping": {
"source_name": "name",
"target_name": "name"
}
},
{
"label": "created",
"source": ["source_name"],
"target": ["target_id"],
"input": {
"type": "file",
"path": "example/file/edge_created.json",
"format": "JSON",
"date_format": "yyyy-MM-dd"
},
"field_mapping": {
"source_name": "name"
}
}
]
}
4.4 执行命令导入
sh bin/hugegraph-loader.sh -g hugegraph -f example/file/struct.json -s example/file/schema.groovy
导入结束后,会出现类似如下统计信息:
vertices/edges has been loaded this time : 8/6
--------------------------------------------------
count metrics
input read success : 14
input read failure : 0
vertex parse success : 8
vertex parse failure : 0
vertex insert success : 8
vertex insert failure : 0
edge parse success : 6
edge parse failure : 0
edge insert success : 6
edge insert failure : 0
4.5 使用 docker 导入
4.5.1 使用 docker exec 直接导入数据
4.5.1.1 数据准备
如果仅仅尝试使用 loader, 我们可以使用内置的 example 数据集进行导入,无需自己额外准备数据
如果使用自定义的数据,则在使用 loader 导入数据之前,我们需要将数据复制到容器内部。
首先我们可以根据 4.1-4.3 的步骤准备数据,将准备好的数据通过 docker cp
复制到 loader 容器内部。
假设我们已经按照上述的步骤准备好了对应的数据集,存放在 hugegraph-dataset
文件夹下,文件结构如下:
tree -f hugegraph-dataset/
hugegraph-dataset
├── hugegraph-dataset/edge_created.json
├── hugegraph-dataset/edge_knows.json
├── hugegraph-dataset/schema.groovy
├── hugegraph-dataset/struct.json
├── hugegraph-dataset/vertex_person.csv
└── hugegraph-dataset/vertex_software.txt
将文件复制到容器内部
docker cp hugegraph-dataset loader:/loader/dataset
docker exec -it loader ls /loader/dataset
edge_created.json edge_knows.json schema.groovy struct.json vertex_person.csv vertex_software.txt
4.5.1.2 数据导入
以内置的 example 数据集为例,我们可以使用以下的命令对数据进行导入。
如果需要导入自己准备的数据集,则只需要修改 -f
配置脚本的路径 以及 -s
schema 文件路径即可。
其他的参数可以参照 3.4.1 参数说明
docker exec -it loader bin/hugegraph-loader.sh -g hugegraph -f example/file/struct.json -s example/file/schema.groovy -h server -p 8080
如果导入用户自定义的数据集,按照刚才的例子,则使用:
docker exec -it loader bin/hugegraph-loader.sh -g hugegraph -f /loader/dataset/struct.json -s /loader/dataset/schema.groovy -h server -p 8080
如果
loader
和server
位于同一 docker 网络,则可以指定-h {server_container_name}
, 否则需要指定server
的宿主机的 ip (在我们的例子中,server_container_name
为server
).
然后我们可以观察到结果:
HugeGraphLoader worked in NORMAL MODE
vertices/edges loaded this time : 8/6
--------------------------------------------------
count metrics
input read success : 14
input read failure : 0
vertex parse success : 8
vertex parse failure : 0
vertex insert success : 8
vertex insert failure : 0
edge parse success : 6
edge parse failure : 0
edge insert success : 6
edge insert failure : 0
--------------------------------------------------
meter metrics
total time : 0.199s
read time : 0.046s
load time : 0.153s
vertex load time : 0.077s
vertex load rate(vertices/s) : 103
edge load time : 0.112s
edge load rate(edges/s) : 53
也可以使用 curl
或者 hubble
观察导入结果,此处以 curl
为例:
> curl "http://localhost:8080/graphs/hugegraph/graph/vertices" | gunzip
{"vertices":[{"id":1,"label":"software","type":"vertex","properties":{"name":"lop","lang":"java","price":328.0}},{"id":2,"label":"software","type":"vertex","properties":{"name":"ripple","lang":"java","price":199.0}},{"id":"1:tom","label":"person","type":"vertex","properties":{"name":"tom"}},{"id":"1:josh","label":"person","type":"vertex","properties":{"name":"josh","age":32,"city":"Beijing"}},{"id":"1:marko","label":"person","type":"vertex","properties":{"name":"marko","age":29,"city":"Beijing"}},{"id":"1:peter","label":"person","type":"vertex","properties":{"name":"peter","age":35,"city":"Shanghai"}},{"id":"1:vadas","label":"person","type":"vertex","properties":{"name":"vadas","age":27,"city":"Hongkong"}},{"id":"1:li,nary","label":"person","type":"vertex","properties":{"name":"li,nary","age":26,"city":"Wu,han"}}]}
如果想检查边的导入结果,可以使用 curl "http://localhost:8080/graphs/hugegraph/graph/edges" | gunzip
4.5.2 进入 docker 容器进行导入
除了直接使用 docker exec
导入数据,我们也可以进入容器进行数据导入,基本流程与 4.5.1 相同
使用 docker exec -it loader bash
进入容器内部,并执行命令
sh bin/hugegraph-loader.sh -g hugegraph -f example/file/struct.json -s example/file/schema.groovy -h server -p 8080
执行的结果如 4.5.1 所示
4.6 使用 spark-loader 导入
Spark 版本:Spark 3+,其他版本未测试。 HugeGraph Toolchain 版本:toolchain-1.0.0
spark-loader
的参数分为两部分,注意:因二者参数名缩写存在重合部分,请使用参数全称。两种参数之间无需保证先后顺序。
- hugegraph 参数(参考:hugegraph-loader 参数说明 )
- Spark 任务提交参数(参考:Submitting Applications)
示例:
sh bin/hugegraph-spark-loader.sh --master yarn \
--deploy-mode cluster --name spark-hugegraph-loader --file ./hugegraph.json \
--username admin --token admin --host xx.xx.xx.xx --port 8093 \
--graph graph-test --num-executors 6 --executor-cores 16 --executor-memory 15g
3 - HugeGraph-Hubble Quick Start
1 HugeGraph-Hubble 概述
特别注意: 当前版本的 Hubble 还没有添加 Auth/Login 相关界面和接口和单独防护, 在下一个 Release 版 (> 1.5) 会加入, 请留意避免把它暴露在公网环境或不受信任的网络中,以免引起相关 SEC 问题 (另外也可以使用 IP & 端口白名单 + HTTPS)
HugeGraph-Hubble 是 HugeGraph 的一站式可视化分析平台,平台涵盖了从数据建模,到数据快速导入, 再到数据的在线、离线分析、以及图的统一管理的全过程,实现了图应用的全流程向导式操作,旨在提升用户的使用流畅度, 降低用户的使用门槛,提供更为高效易用的使用体验。
平台主要包括以下模块:
图管理
图管理模块通过图的创建,连接平台与图数据,实现多图的统一管理,并实现图的访问、编辑、删除、查询操作。
元数据建模
元数据建模模块通过创建属性库,顶点类型,边类型,索引类型,实现图模型的构建与管理,平台提供两种模式,列表模式和图模式,可实时展示元数据模型,更加直观。同时还提供了跨图的元数据复用功能,省去相同元数据繁琐的重复创建过程,极大地提升建模效率,增强易用性。
图分析
通过输入图遍历语言 Gremlin 可实现图数据的高性能通用分析,并提供顶点的定制化多维路径查询等功能,提供 3 种图结果展示方式,包括:图形式、表格形式、Json 形式,多维度展示数据形态,满足用户使用的多种场景需求。提供运行记录及常用语句收藏等功能,实现图操作的可追溯,以及查询输入的复用共享,快捷高效。支持图数据的导出,导出格式为 Json 格式。
任务管理
对于需要遍历全图的 Gremlin 任务,索引的创建与重建等耗时较长的异步任务,平台提供相应的任务管理功能,实现异步任务的统一的管理与结果查看。
数据导入 (BETA)
注: 数据导入功能目前适合初步试用,正式数据导入请使用 hugegraph-loader, 性能/稳定性/功能全面许多
数据导入是将用户的业务数据转化为图的顶点和边并插入图数据库中,平台提供了向导式的可视化导入模块,通过创建导入任务, 实现导入任务的管理及多个导入任务的并行运行,提高导入效能。进入导入任务后,只需跟随平台步骤提示,按需上传文件,填写内容, 就可轻松实现图数据的导入过程,同时支持断点续传,错误重试机制等,降低导入成本,提升效率。
2 部署
有三种方式可以部署hugegraph-hubble
- 使用 docker (便于测试)
- 下载 toolchain 二进制包
- 源码编译
2.1 使用 Docker (便于测试)
特别注意: docker 模式下,若 hubble 和 server 在同一宿主机,hubble 页面中设置 server 的
hostname
不能设置为localhost/127.0.0.1
,因这会指向 hubble 容器内部而非宿主机,导致无法连接到 server.若 hubble 和 server 在同一 docker 网络下,推荐直接使用
container_name
(如下例的server
) 作为主机名。或者也可以使用 宿主机 IP 作为主机名,此时端口号为宿主机给 server 配置的端口
我们可以使用 docker run -itd --name=hubble -p 8088:8088 hugegraph/hubble:1.5.0
快速启动 hubble.
或者使用 docker-compose 启动 hubble,另外如果 hubble 和 server 在同一个 docker 网络下,可以使用 server 的 contain_name 进行访问,而不需要宿主机的 ip
使用docker-compose up -d
,docker-compose.yml
如下:
version: '3'
services:
server:
image: hugegraph/hugegraph:1.5.0
container_name: server
ports:
- 8080:8080
hubble:
image: hugegraph/hubble:1.5.0
container_name: hubble
ports:
- 8088:8088
注意:
hugegraph-hubble
的 docker 镜像是一个便捷发布版本,用于快速测试试用 hubble,并非ASF 官方发布物料包的方式。你可以从 ASF Release Distribution Policy 中得到更多细节。生产环境推荐使用
release tag
(如1.5.0
) 稳定版。使用latest
tag 默认对应 master 最新代码。
2.2 下载 toolchain 二进制包
hubble
项目在toolchain
项目中,首先下载toolchain
的 tar 包
wget https://downloads.apache.org/incubator/hugegraph/{version}/apache-hugegraph-toolchain-incubating-{version}.tar.gz
tar -xvf apache-hugegraph-toolchain-incubating-{version}.tar.gz
cd apache-hugegraph-toolchain-incubating-{version}.tar.gz/apache-hugegraph-hubble-incubating-{version}
运行hubble
bin/start-hubble.sh
随后我们可以看到
starting HugeGraphHubble ..............timed out with http status 502
2023-08-30 20:38:34 [main] [INFO ] o.a.h.HugeGraphHubble [] - Starting HugeGraphHubble v1.0.0 on cpu05 with PID xxx (~/apache-hugegraph-toolchain-incubating-1.0.0/apache-hugegraph-hubble-incubating-1.0.0/lib/hubble-be-1.0.0.jar started by $USER in ~/apache-hugegraph-toolchain-incubating-1.0.0/apache-hugegraph-hubble-incubating-1.0.0)
...
2023-08-30 20:38:38 [main] [INFO ] c.z.h.HikariDataSource [] - hugegraph-hubble-HikariCP - Start completed.
2023-08-30 20:38:41 [main] [INFO ] o.a.c.h.Http11NioProtocol [] - Starting ProtocolHandler ["http-nio-0.0.0.0-8088"]
2023-08-30 20:38:41 [main] [INFO ] o.a.h.HugeGraphHubble [] - Started HugeGraphHubble in 7.379 seconds (JVM running for 8.499)
然后使用浏览器访问 ip:8088
可看到hubble
页面,通过bin/stop-hubble.sh
则可以停止服务
2.3 源码编译
注意: 目前已在 hugegraph-hubble/hubble-be/pom.xml
中引入插件 frontend-maven-plugin
,编译 hubble 时不需要用户本地环境提前安装 Nodejs V16.x
与 yarn
环境,可直接按下述步骤执行
下载 toolchain 源码包
git clone https://github.com/apache/hugegraph-toolchain.git
编译hubble
, 它依赖 loader 和 client, 编译时需提前构建这些依赖 (后续可跳)
cd hugegraph-toolchain
sudo pip install -r hugegraph-hubble/hubble-dist/assembly/travis/requirements.txt
mvn install -pl hugegraph-client,hugegraph-loader -am -Dmaven.javadoc.skip=true -DskipTests -ntp
cd hugegraph-hubble
mvn -e package -Dmaven.javadoc.skip=true -Dmaven.test.skip=true -ntp
cd apache-hugegraph-hubble-incubating*
启动hubble
bin/start-hubble.sh -d
3 平台使用流程
平台的模块使用流程如下:

4 平台使用说明
4.1 图管理
4.1.1 图创建
图管理模块下,点击【创建图】,通过填写图 ID、图名称、主机名、端口号、用户名、密码的信息,实现多图的连接。
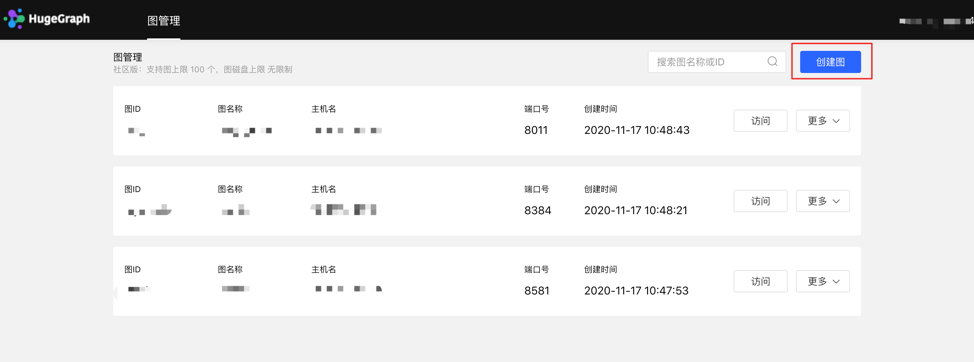
创建图填写内容如下:
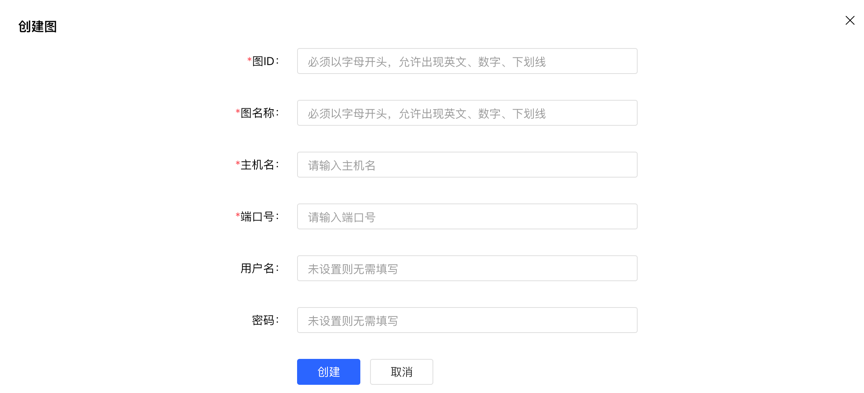
注意:如果使用 docker 启动
hubble
,且server
和hubble
位于同一宿主机,不能直接使用localhost/127.0.0.1
作为主机名。如果hubble
和server
在同一 docker 网络下,则可以直接使用 container_name 作为主机名,端口则为 8080。或者也可以使用宿主机 ip 作为主机名,此时端口为宿主机为 server 配置的端口
4.1.2 图访问
实现图空间的信息访问,进入后,可进行图的多维查询分析、元数据管理、数据导入、算法分析等操作。

4.1.3 图管理
- 用户通过对图的概览、搜索以及单图的信息编辑与删除,实现图的统一管理。
- 搜索范围:可对图名称和 ID 进行搜索。
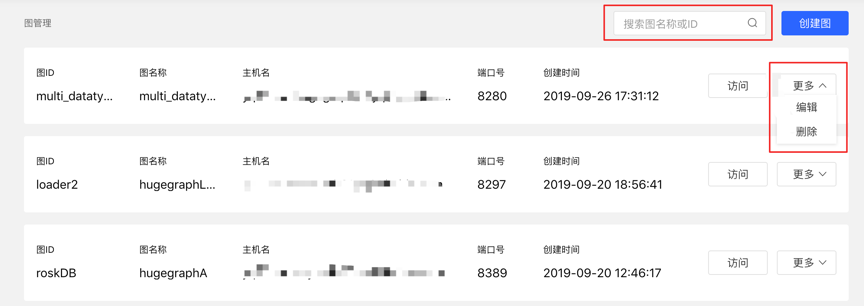
4.2 元数据建模(列表 + 图模式)
4.2.1 模块入口
左侧导航处:
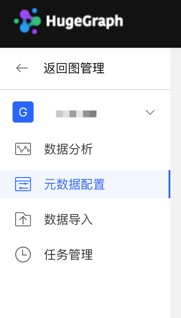
4.2.2 属性类型
4.2.2.1 创建
- 填写或选择属性名称、数据类型、基数,完成属性的创建。
- 创建的属性可作为顶点类型和边类型的属性。
列表模式:
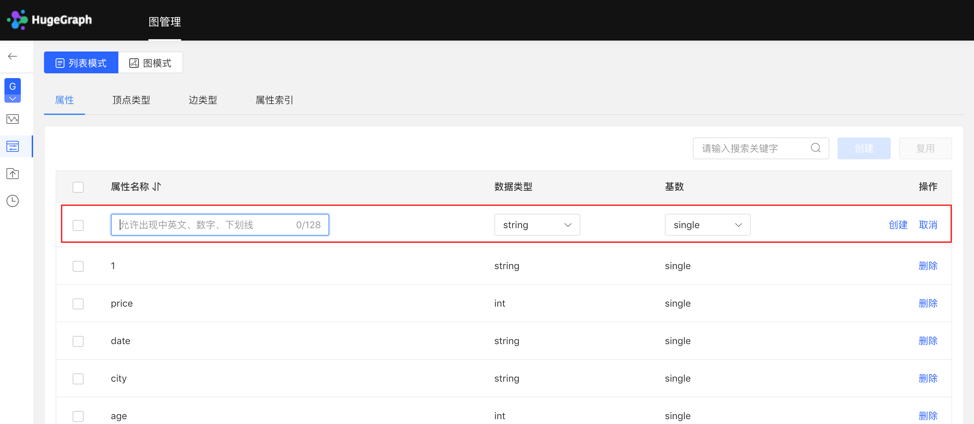
图模式:
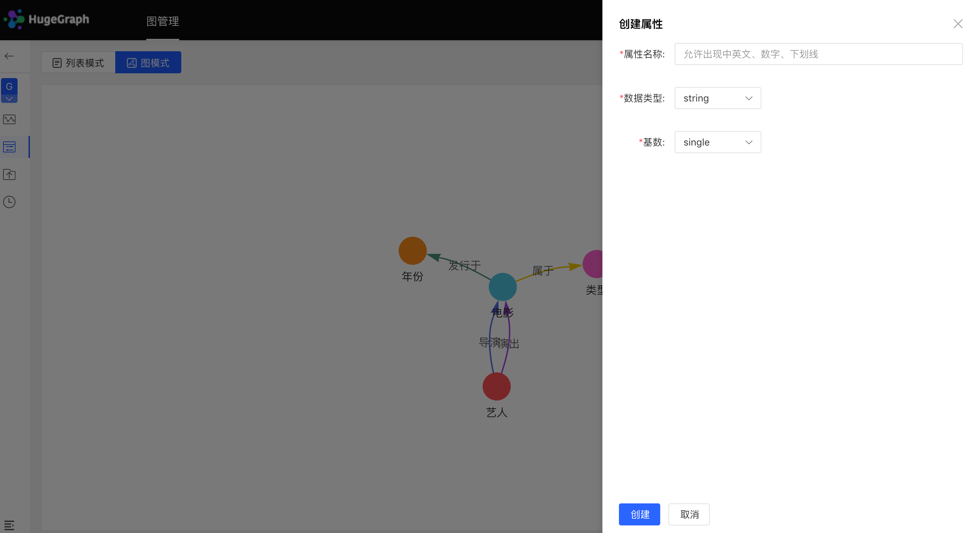
4.2.2.2 复用
- 平台提供【复用】功能,可直接复用其他图的元数据。
- 选择需要复用的图 ID,继续选择需要复用的属性,之后平台会进行是否冲突的校验,通过后,可实现元数据的复用。
选择复用项:
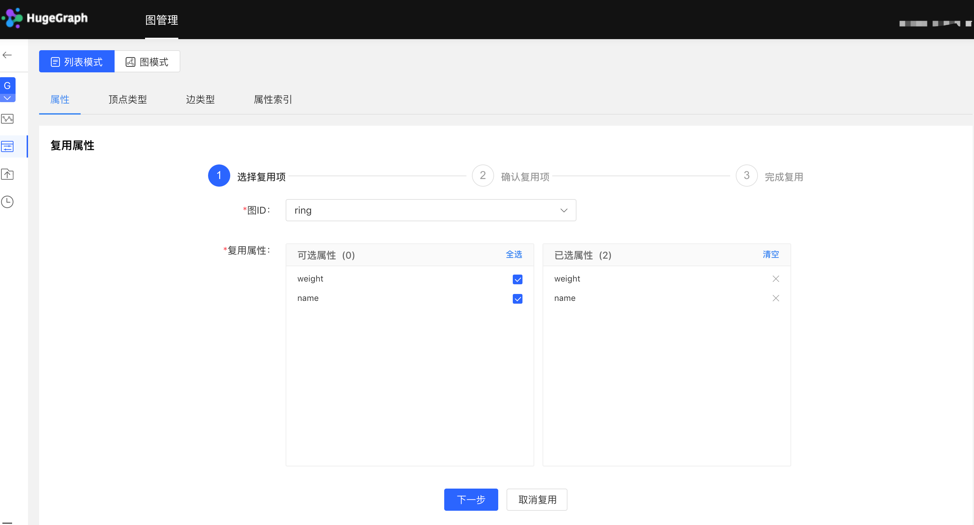
校验复用项:
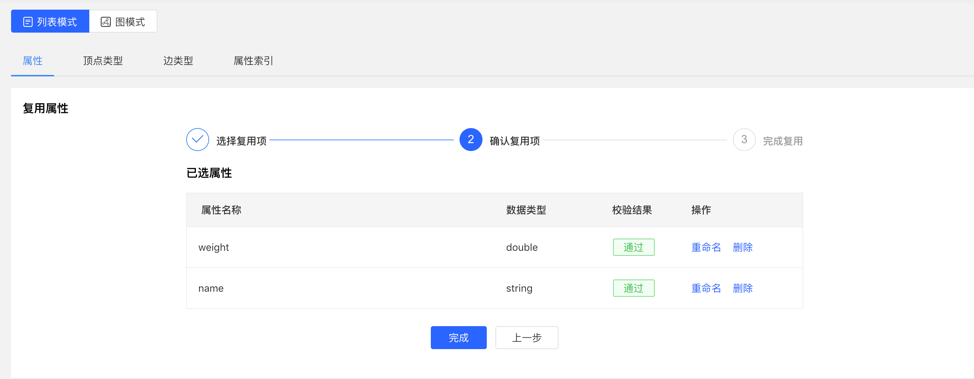
4.2.2.3 管理
- 在属性列表中可进行单条删除或批量删除操作。
4.2.3 顶点类型
4.2.3.1 创建
- 填写或选择顶点类型名称、ID 策略、关联属性、主键属性,顶点样式、查询结果中顶点下方展示的内容,以及索引的信息:包括是否创建类型索引,及属性索引的具体内容,完成顶点类型的创建。
列表模式:
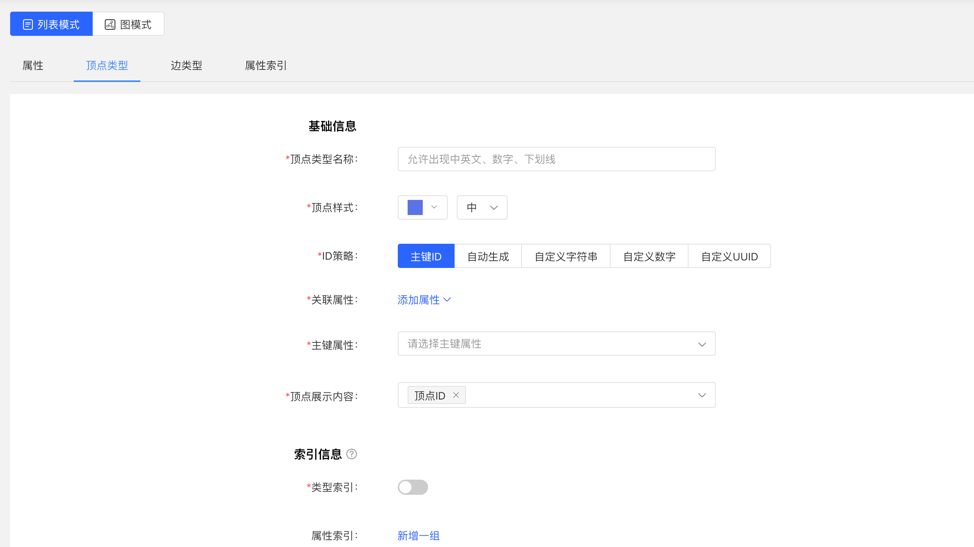
图模式:
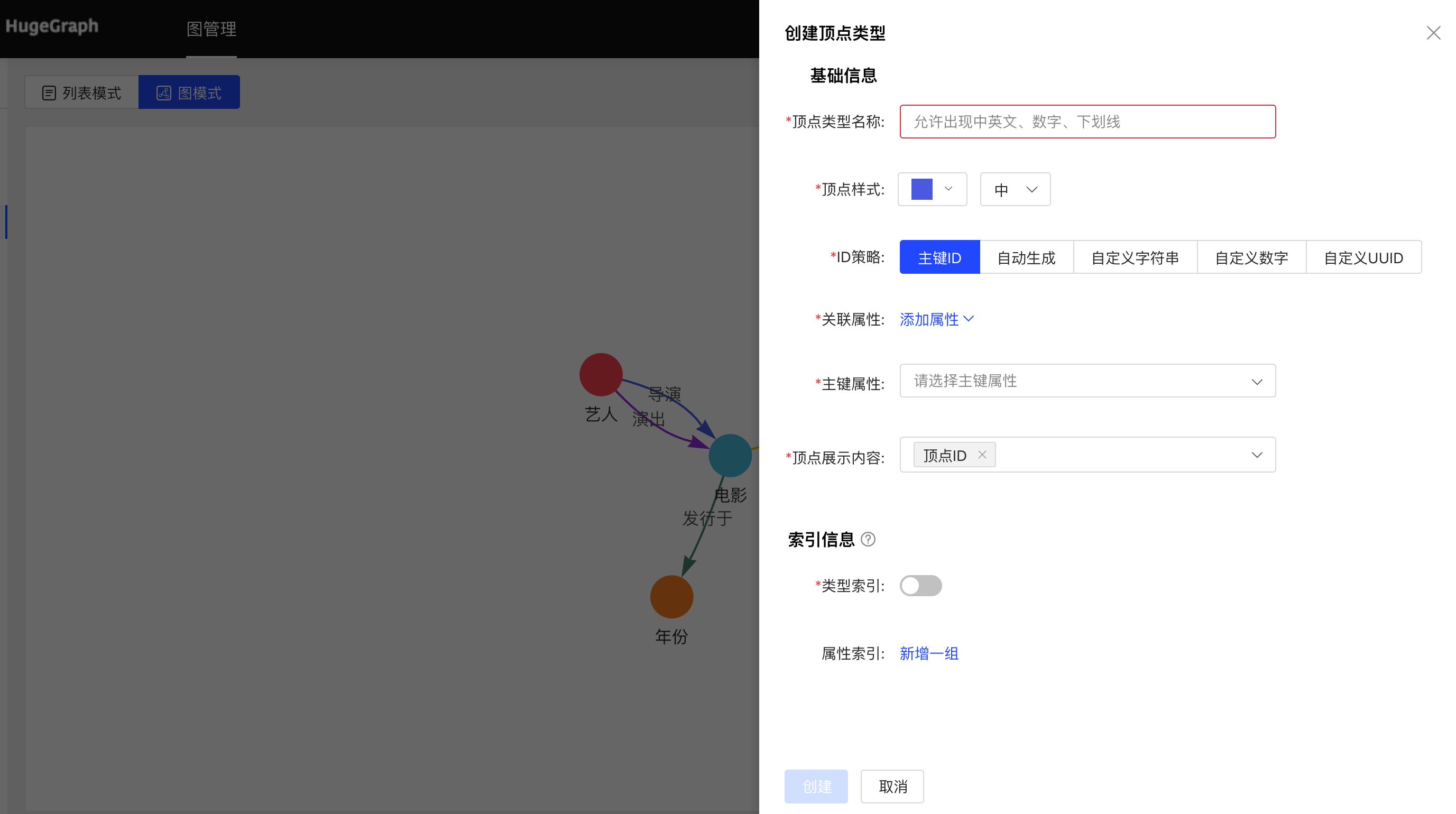
4.2.3.2 复用
- 顶点类型的复用,会将此类型关联的属性和属性索引一并复用。
- 复用功能使用方法类似属性的复用,见 3.2.2.2。
4.2.3.3 管理
可进行编辑操作,顶点样式、关联类型、顶点展示内容、属性索引可编辑,其余不可编辑。
可进行单条删除或批量删除操作。
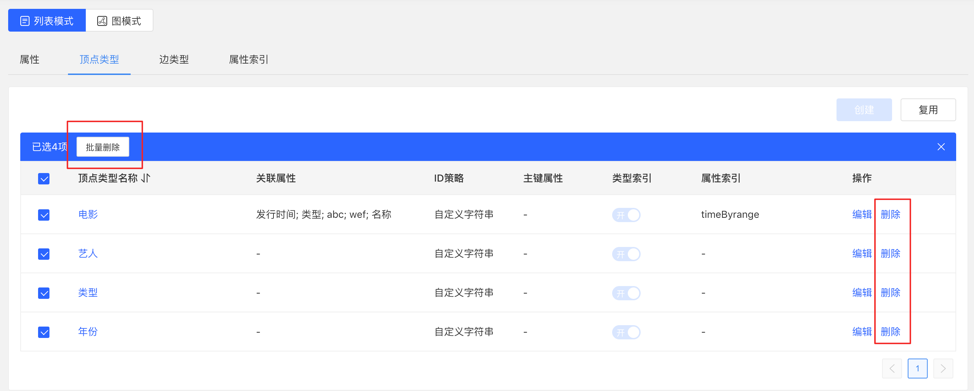
4.2.4 边类型
4.2.4.1 创建
- 填写或选择边类型名称、起点类型、终点类型、关联属性、是否允许多次连接、边样式、查询结果中边下方展示的内容,以及索引的信息:包括是否创建类型索引,及属性索引的具体内容,完成边类型的创建。
列表模式:
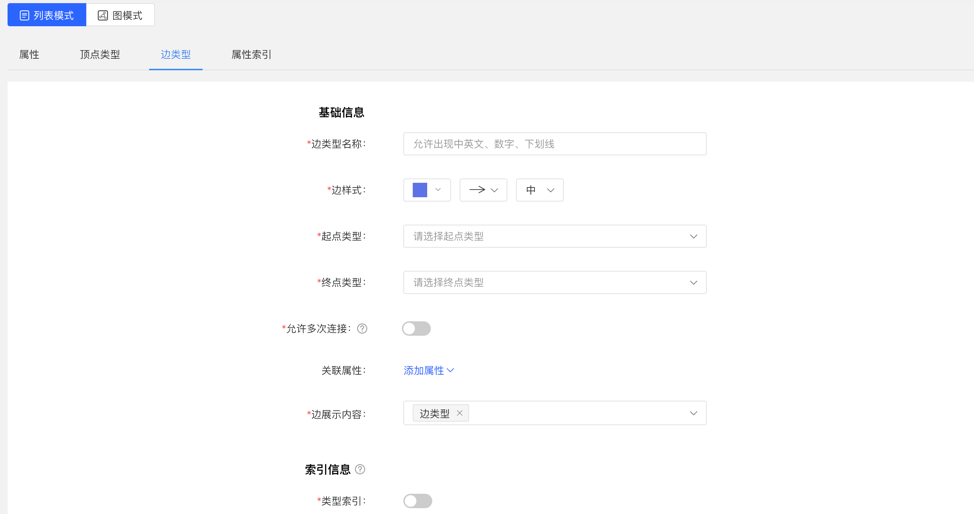
图模式:
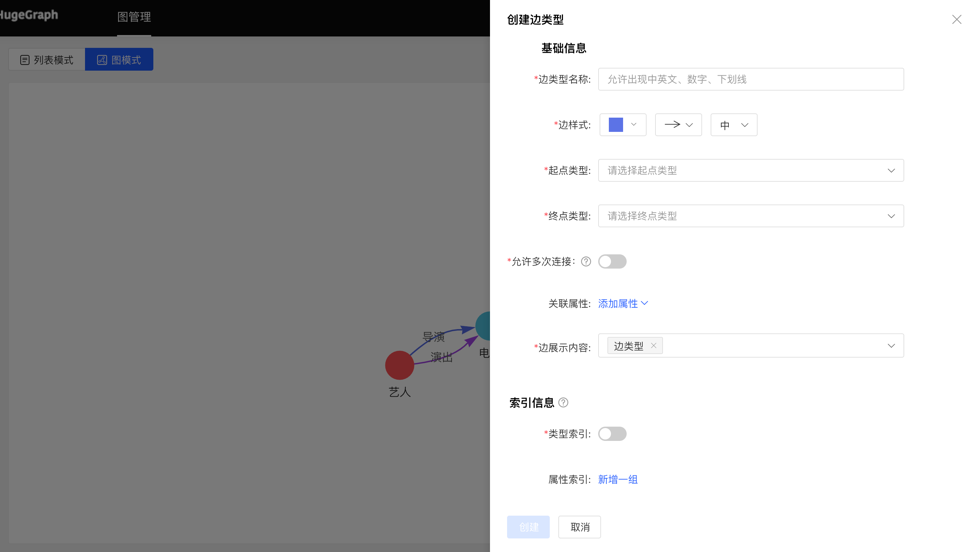
4.2.4.2 复用
- 边类型的复用,会将此类型的起点类型、终点类型、关联的属性和属性索引一并复用。
- 复用功能使用方法类似属性的复用,见 3.2.2.2。
4.2.4.3 管理
- 可进行编辑操作,边样式、关联属性、边展示内容、属性索引可编辑,其余不可编辑,同顶点类型。
- 可进行单条删除或批量删除操作。
4.2.5 索引类型
展示顶点类型和边类型的顶点索引和边索引。
4.3 数据导入
注意:目前推荐使用 hugegraph-loader 进行正式数据导入,hubble 内置的导入用来做测试和简单上手
数据导入的使用流程如下:

4.3.1 模块入口
左侧导航处:
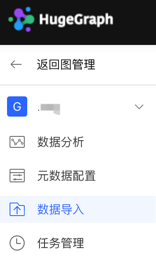
4.3.2 创建任务
- 填写任务名称和备注(非必填),可以创建导入任务。
- 可创建多个导入任务,并行导入。
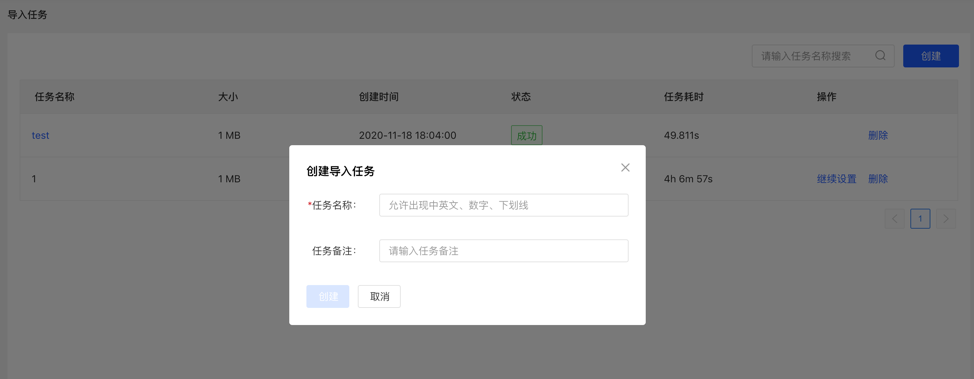
4.3.3 上传文件
- 上传需要构图的文件,目前支持的格式为 CSV,后续会不断更新。
- 可同时上传多个文件。
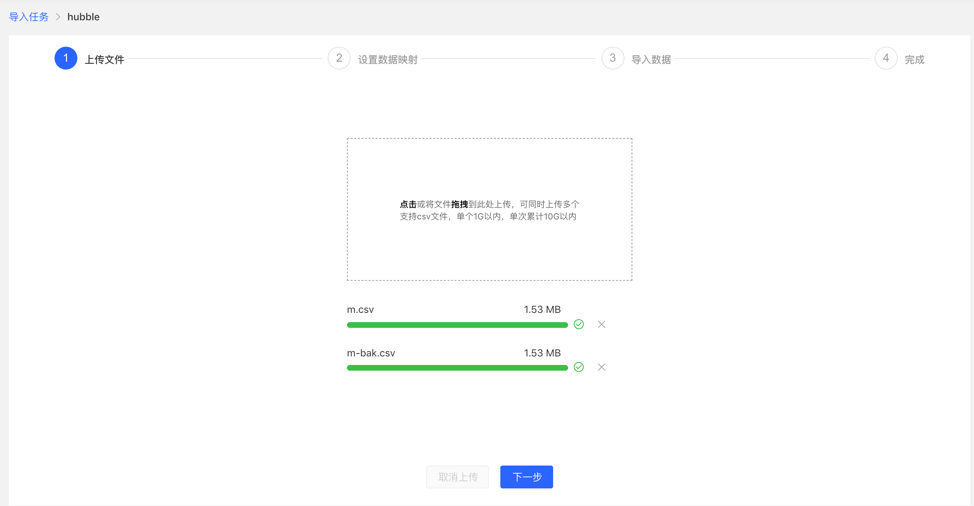
4.3.4 设置数据映射
对上传的文件分别设置数据映射,包括文件设置和类型设置
文件设置:勾选或填写是否包含表头、分隔符、编码格式等文件本身的设置内容,均设置默认值,无需手动填写
类型设置:
顶点映射和边映射:
【顶点类型】 :选择顶点类型,并为其 ID 映射上传文件中列数据;
【边类型】:选择边类型,为其起点类型和终点类型的 ID 列映射上传文件的列数据;
映射设置:为选定的顶点类型的属性映射上传文件中的列数据,此处,若属性名称与文件的表头名称一致,可自动匹配映射属性,无需手动填选
完成设置后,显示设置列表,方可进行下一步操作,支持映射的新增、编辑、删除操作
设置映射的填写内容:
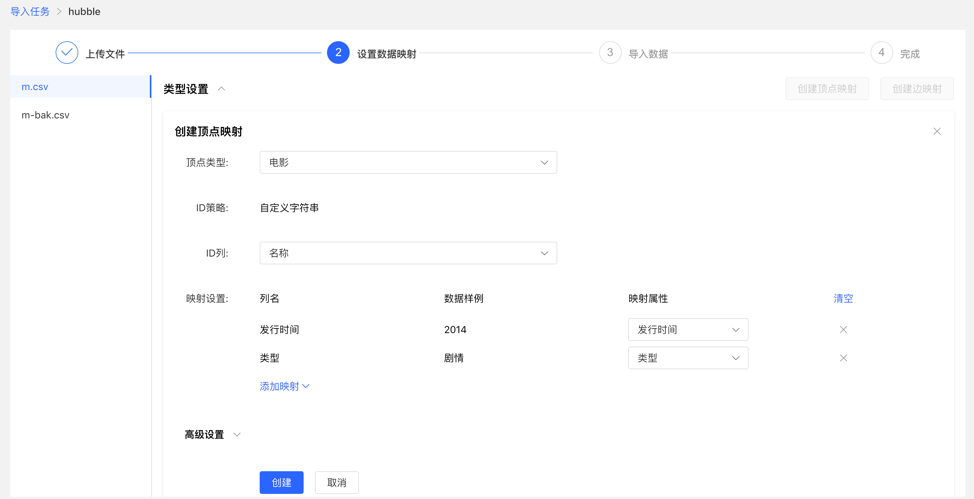
映射列表:
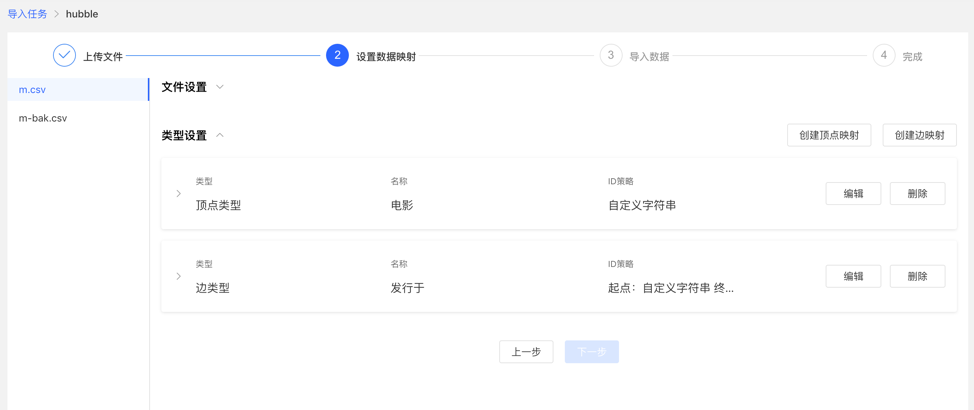
4.3.5 导入数据
导入前需要填写导入设置参数,填写完成后,可开始向图库中导入数据
- 导入设置
- 导入设置参数项如下图所示,均设置默认值,无需手动填写
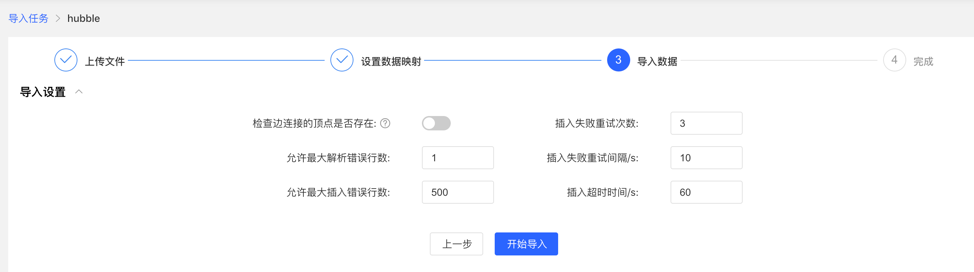
- 导入详情
- 点击开始导入,开始文件的导入任务
- 导入详情中提供每个上传文件设置的映射类型、导入速度、导入的进度、耗时以及当前任务的具体状态,并可对每个任务进行暂停、继续、停止等操作
- 若导入失败,可查看具体原因
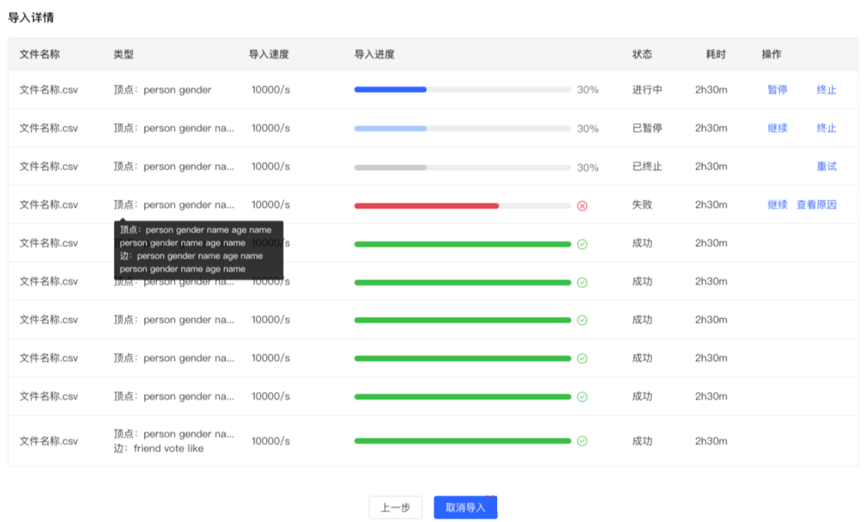
4.4 数据分析
4.4.1 模块入口
左侧导航处:
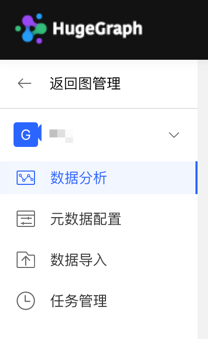
4.4.2 多图切换
通过左侧切换入口,灵活切换多图的操作空间
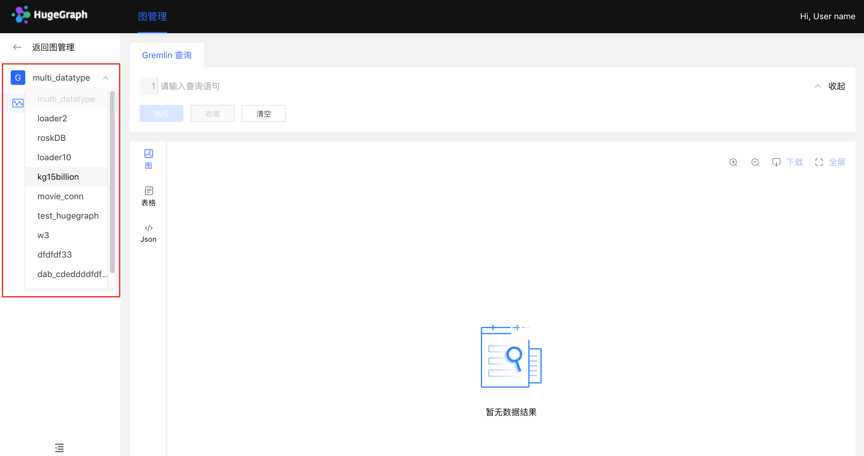
4.4.3 图分析与处理
HugeGraph 支持 Apache TinkerPop3 的图遍历查询语言 Gremlin,Gremlin 是一种通用的图数据库查询语言,通过输入 Gremlin 语句,点击执行,即可执行图数据的查询分析操作,并可实现顶点/边的创建及删除、顶点/边的属性修改等。
Gremlin 查询后,下方为图结果展示区域,提供 3 种图结果展示方式,分别为:【图模式】、【表格模式】、【Json 模式】。
支持缩放、居中、全屏、导出等操作。
【图模式】
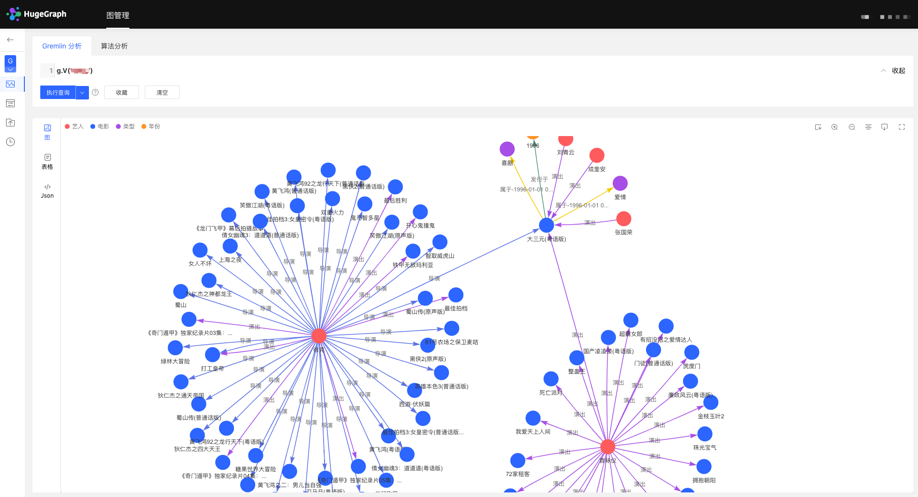
【表格模式】
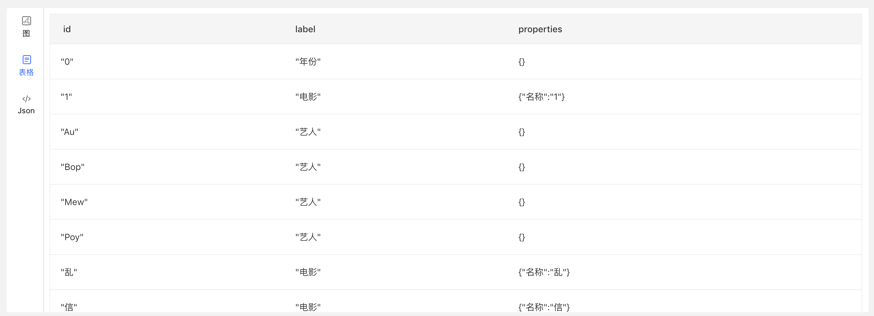
【Json 模式】
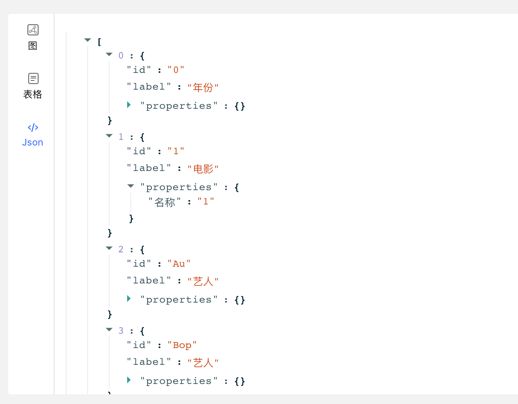
4.4.4 数据详情
点击顶点/边实体,可查看顶点/边的数据详情,包括:顶点/边类型,顶点 ID,属性及对应值,拓展图的信息展示维度,提高易用性。
4.4.5 图结果的多维路径查询
除了全局的查询外,可针对查询结果中的顶点进行深度定制化查询以及隐藏操作,实现图结果的定制化挖掘。
右击顶点,出现顶点的菜单入口,可进行展示、查询、隐藏等操作。
- 展开:点击后,展示与选中点关联的顶点。
- 查询:通过选择与选中点关联的边类型及边方向,在此条件下,再选择其属性及相应筛选规则,可实现定制化的路径展示。
- 隐藏:点击后,隐藏选中点及与之关联的边。
双击顶点,也可展示与选中点关联的顶点。
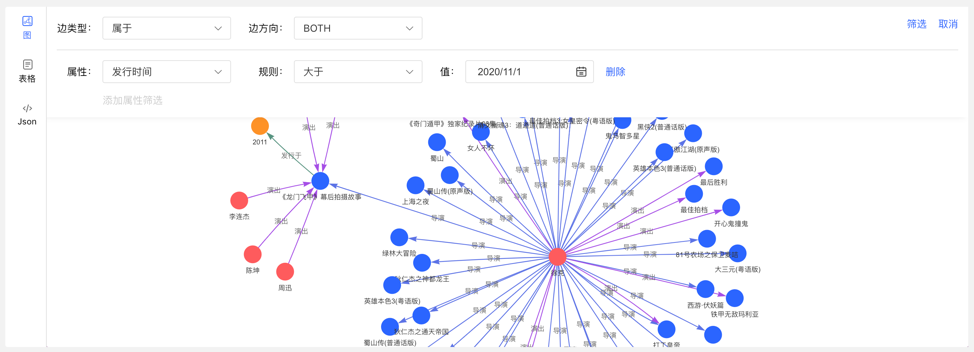
4.4.6 新增顶点/边
4.4.6.1 新增顶点
在图区可通过两个入口,动态新增顶点,如下:
- 点击图区面板,出现添加顶点入口
- 点击右上角的操作栏中的首个图标
通过选择或填写顶点类型、ID 值、属性信息,完成顶点的增加。
入口如下:
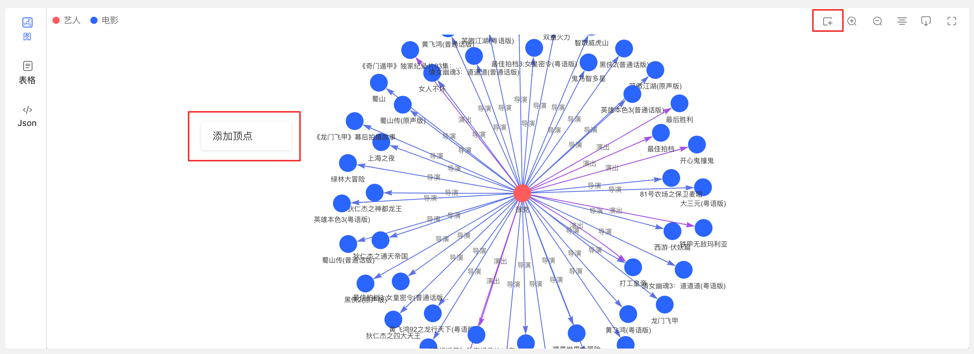
添加顶点内容如下:
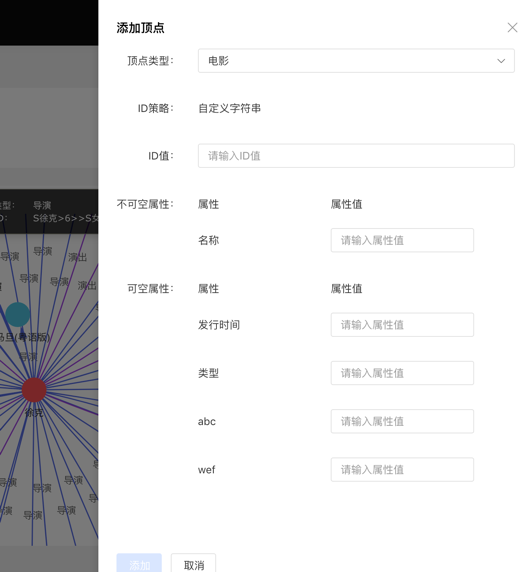
4.4.6.2 新增边
右击图结果中的顶点,可增加该点的出边或者入边。
4.4.7 执行记录与收藏的查询
- 图区下方记载每次查询记录,包括:查询时间、执行类型、内容、状态、耗时、以及【收藏】和【加载】操作,实现图执行的全方位记录,有迹可循,并可对执行内容快速加载复用
- 提供语句的收藏功能,可对常用语句进行收藏操作,方便高频语句快速调用
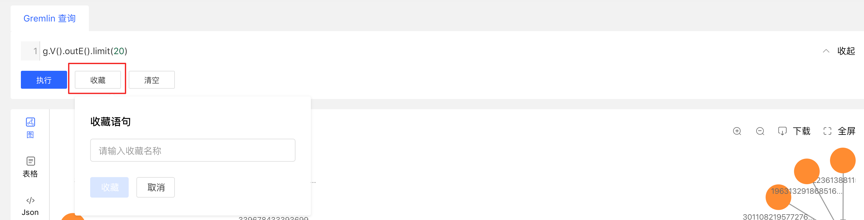
4.5 任务管理
4.5.1 模块入口
左侧导航处:
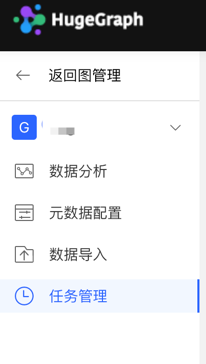
4.5.2 任务管理
- 提供异步任务的统一的管理与结果查看,异步任务包括 4 类,分别为:
- gremlin:Gremlin 任务务
- algorithm:OLAP 算法任务务
- remove_schema:删除元数据
- rebuild_index:重建索引
- 列表显示当前图的异步任务信息,包括:任务 ID,任务名称,任务类型,创建时间,耗时,状态,操作,实现对异步任务的管理。
- 支持对任务类型和状态进行筛选
- 支持搜索任务 ID 和任务名称
- 可对异步任务进行删除或批量删除操作
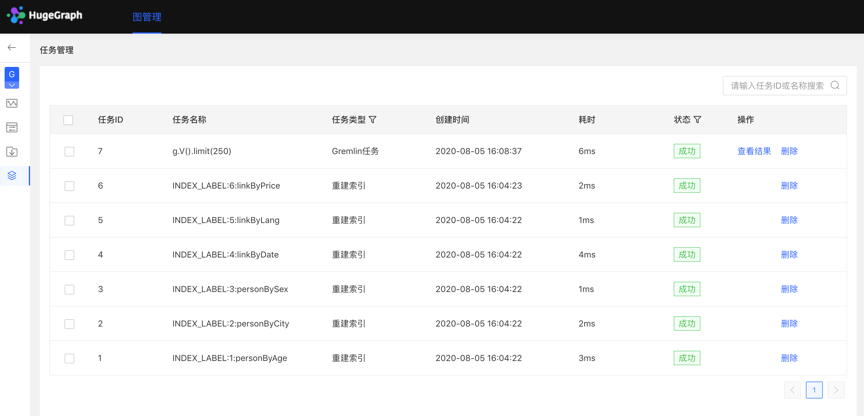
4.5.3 Gremlin 异步任务
1.创建任务
- 数据分析模块,目前支持两种 Gremlin 操作,Gremlin 查询和 Gremlin 任务;若用户切换到 Gremlin 任务,点击执行后,在异步任务中心会建立一条异步任务; 2.任务提交
- 任务提交成功后,图区部分返回提交结果和任务 ID 3.任务详情
- 提供【查看】入口,可跳转到任务详情查看当前任务具体执行情况跳转到任务中心后,直接显示当前执行的任务行
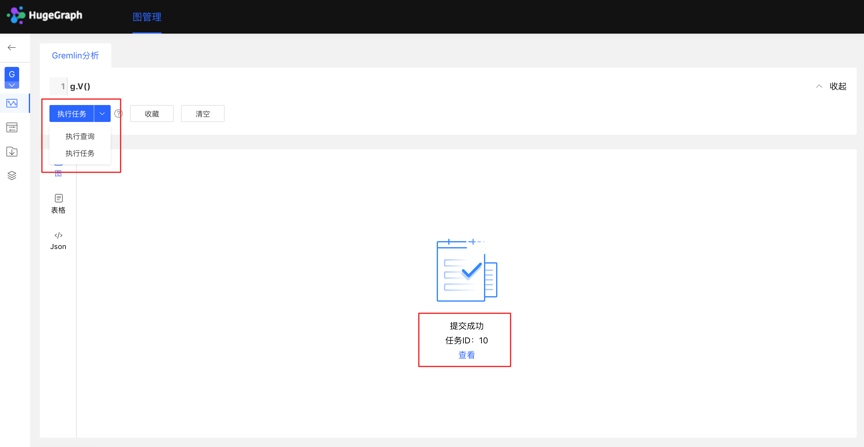
点击查看入口,跳转到任务管理列表,如下:
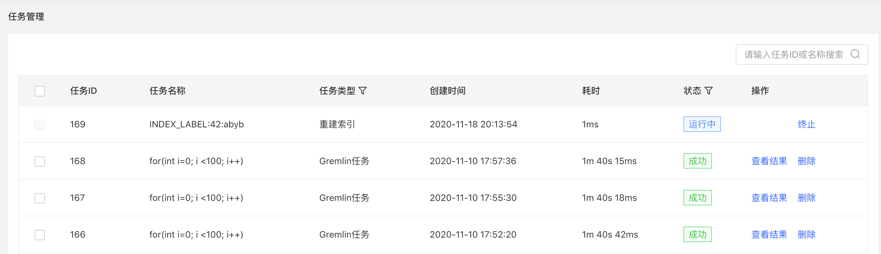
4.查看结果
- 结果通过 json 形式展示
4.5.4 OLAP 算法任务
Hubble 上暂未提供可视化的 OLAP 算法执行,可调用 RESTful API 进行 OLAP 类算法任务,在任务管理中通过 ID 找到相应任务,查看进度与结果等。
4.5.5 删除元数据、重建索引
1.创建任务
- 在元数据建模模块中,删除元数据时,可建立删除元数据的异步任务
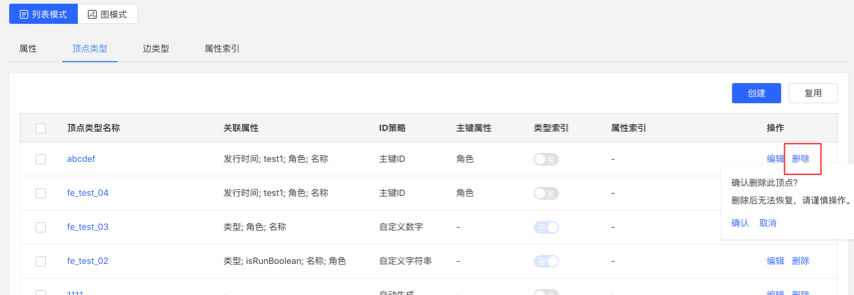
- 在编辑已有的顶点/边类型操作中,新增索引时,可建立创建索引的异步任务
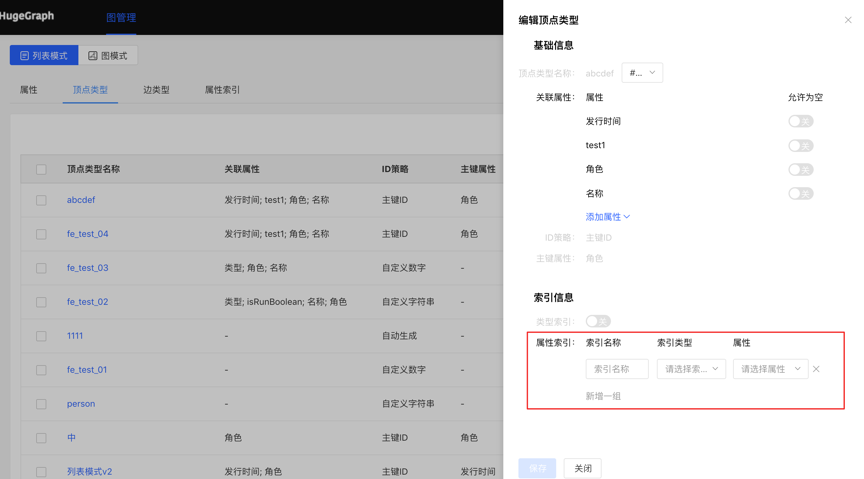
2.任务详情
- 确认/保存后,可跳转到任务中心查看当前任务的详情
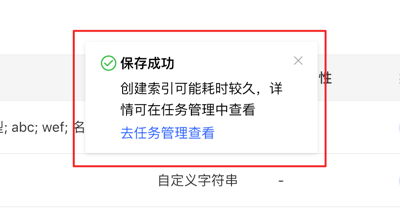
4 - HugeGraph-AI Quick Start
1 HugeGraph-AI 概述
hugegraph-ai 旨在探索 HugeGraph 与人工智能(AI)的融合,包括与大模型结合的应用,与图机器学习组件的集成等,为开发者在项目中利用 HugeGraph 的 AI 能力提供全面支持。
2 环境要求
- python 3.9+ (better to use
3.10
) - hugegraph-server 1.3+
3 准备工作
- 启动HugeGraph数据库,可以通过 Docker/Binary Package 运行它。
请参阅详细文档以获取更多指导 - 克隆项目
git clone https://github.com/apache/incubator-hugegraph-ai.git
- 安装 hugegraph-python-client 和 hugegraph_llm
cd ./incubator-hugegraph-ai # better to use virtualenv (source venv/bin/activate) pip install ./hugegraph-python-client pip install -r ./hugegraph-llm/requirements.txt
- 进入项目目录
cd ./hugegraph-llm/src
- 启动 Graph RAG 的 gradio 交互 demo,可以使用以下命令运行,启动后打开 http://127.0.0.1:8001默认主机为
python3 -m hugegraph_llm.demo.rag_demo.app
0.0.0.0
,端口为8001
。您可以通过传递命令行参数--host
和--port
来更改它们。python3 -m hugegraph_llm.demo.rag_demo.app --host 127.0.0.1 --port 18001
- 启动 Text2Gremlin 的 gradio 交互演示,可以使用以下命令运行,启动后打开 http://127.0.0.1:8002 ,您还可以按上述方式更改默认主机
0.0.0.0
和端口8002
。(🚧ing)python3 -m hugegraph_llm.demo.gremlin_generate_web_demo
- 在运行演示程序后,配置文件文件将被删除。
.env
将自动生成在hugegraph-llm/.env
路径下。此外,还有一个与 prompt 相关的配置文件config_prompt.yaml
。也会在hugegraph-llm/src/hugegraph_llm/resources/demo/config_prompt.yaml
路径下生成。 您可以在页面上修改内容,触发相应功能后会自动保存到配置文件中。你也可以直接修改文件而无需重启应用程序;只需刷新页面即可加载最新的更改。 (可选)要重新生成配置文件,您可以将config.generate
与-u
或--update
一起使用。python3 -m hugegraph_llm.config.generate --update
- (可选)您可以使用 hugegraph-hubble 来访问图形数据,可以通过 Docker/Docker-Compose 运行它以获得指导。 (Hubble 是一个图形分析仪表板,包括数据加载/模式管理/图形遍历/显示)。
- (可选)离线下载 NLTK 停用词
python ./hugegraph_llm/operators/common_op/nltk_helper.py
4 示例
4.1 通过 LLM 在 HugeGraph 中构建知识图谱
4.1.1 通过 gradio 交互式界面构建知识图谱
参数描述:
- Docs:
- text: 从纯文本建立 rag 索引
- file: 上传文件:TXT 或 .docx(可同时选择多个文件)
- Schema:(接受2种类型)
- 用户定义模式( JSON 格式,遵循模板来修改它)
- 指定 HugeGraph 图实例的名称,它将自动从中获取模式(如 “hugegraph”)
- Graph extract head: 用户自定义的图提取提示
- 如果已经存在图数据,你应该点击 “Rebuild vid Index” 来更新索引
4.1.2 通过代码构建知识图谱
KgBuilder
类用于构建知识图谱。下面是使用过程:
- 初始化:
KgBuilder
类使用语言模型的实例进行初始化。这可以从LLMs
类中获得。 初始化LLMs
实例,获取LLM
,然后创建一个任务实例KgBuilder
用于图的构建。KgBuilder
定义了多个运算符,用户可以根据需要自由组合它们。(提示:print_result()
可以在控制台中打印出每一步的结果,而不会影响整个执行逻辑)from hugegraph_llm.models.llms.init_llm import LLMs from hugegraph_llm.operators.kg_construction_task import KgBuilder TEXT = "" builder = KgBuilder(LLMs().get_llm()) ( builder .import_schema(from_hugegraph="talent_graph").print_result() .chunk_split(TEXT).print_result() .extract_info(extract_type="property_graph").print_result() .commit_to_hugegraph() .run() )
- 导入架构:
import_schema
方法用于从源导入架构。源可以是 HugeGraph 实例、用户定义的模式或提取结果。可以链接print_result
方法来打印结果。# Import schema from a HugeGraph instance builder.import_schema(from_hugegraph="xxx").print_result() # Import schema from an extraction result builder.import_schema(from_extraction="xxx").print_result() # Import schema from user-defined schema builder.import_schema(from_user_defined="xxx").print_result()
- Chunk Split :
chunk_split
方法用于将输入文本分割为块。文本应该作为字符串参数传递给方法。# Split the input text into documents builder.chunk_split(TEXT, split_type="document").print_result() # Split the input text into paragraphs builder.chunk_split(TEXT, split_type="paragraph").print_result() # Split the input text into sentences builder.chunk_split(TEXT, split_type="sentence").print_result()
- 信息抽取:
extract_info
方法用于从文本中提取信息。文本应该作为字符串参数传递给方法。TEXT = "Meet Sarah, a 30-year-old attorney, and her roommate, James, whom she's shared a home with since 2010." # extract property graph from the input text builder.extract_info(extract_type="property_graph").print_result() # extract triples from the input text builder.extract_info(extract_type="property_graph").print_result()
- Commit to HugeGraph :
commit_to_hugegraph
方法用于将构建的知识图谱提交到 HugeGraph 实例。builder.commit_to_hugegraph().print_result()
- Run :
run
方法用于执行链式操作。builder.run()
KgBuilder
类的方法可以链接在一起以执行一系列操作。
4.2 基于 HugeGraph 的检索增强生成(RAG)
RAGPipeline
类用于将 HugeGraph 与大型语言模型集成,以提供检索增强生成功能。下面是使用过程:
- 提取关键字:提取关键字并扩展同义词。
from hugegraph_llm.operators.graph_rag_task import RAGPipeline graph_rag = RAGPipeline() graph_rag.extract_keywords(text="Tell me about Al Pacino.").print_result()
- 根据关键字匹配 Vid::将节点与图中的关键字匹配。
graph_rag.keywords_to_vid().print_result()
- RAG 的查询图:从 HugeGraph 中检索对应的关键词及其多度关联关系。
graph_rag.query_graphdb(max_deep=2, max_items=30).print_result()
- 重新排序搜索结果:根据问题与结果之间的相似性对搜索结果进行重新排序。
graph_rag.merge_dedup_rerank().print_result()
- 合成答案:总结结果并组织语言来回答问题。
graph_rag.synthesize_answer(vector_only_answer=False, graph_only_answer=True).print_result()
- 运行:
run
方法用于执行上述操作。graph_rag.run(verbose=True)
5 - HugeGraph-Client Quick Start
1 HugeGraph-Client 概述
HugeGraph-Client 向 HugeGraph-Server 发出 HTTP 请求,获取并解析 Server 的执行结果。
提供了 Java/Go/Python 版,
用户可以使用 Client-API 编写代码操作 HugeGraph,比如元数据和图数据的增删改查,或者执行 gremlin 语句等。
后文主要是 Java 使用示例 (其他语言 SDK 可参考对应 READEME
页面)
现在已经支持基于 Go 语言的 HugeGraph Client SDK (version >=1.2.0)
2 环境要求
- java 11 (兼容 java 8)
- maven 3.5+
3 使用流程
使用 HugeGraph-Client 的基本步骤如下:
- 新建Eclipse/ IDEA Maven 项目;
- 在 pom 文件中添加 HugeGraph-Client 依赖;
- 创建类,调用 HugeGraph-Client 接口;
详细使用过程见下节完整示例。
4 完整示例
4.1 新建 Maven 工程
可以选择 Eclipse 或者 Intellij Idea 创建工程:
4.2 添加 hugegraph-client 依赖
添加 hugegraph-client 依赖
<dependencies>
<dependency>
<groupId>org.apache.hugegraph</groupId>
<artifactId>hugegraph-client</artifactId>
<!-- Update to the latest release version -->
<version>1.5.0</version>
</dependency>
</dependencies>
注:Graph 所有组件版本号均保持一致
4.3 Example
4.3.1 SingleExample
import java.io.IOException;
import java.util.Iterator;
import java.util.List;
import org.apache.hugegraph.driver.GraphManager;
import org.apache.hugegraph.driver.GremlinManager;
import org.apache.hugegraph.driver.HugeClient;
import org.apache.hugegraph.driver.SchemaManager;
import org.apache.hugegraph.structure.constant.T;
import org.apache.hugegraph.structure.graph.Edge;
import org.apache.hugegraph.structure.graph.Path;
import org.apache.hugegraph.structure.graph.Vertex;
import org.apache.hugegraph.structure.gremlin.Result;
import org.apache.hugegraph.structure.gremlin.ResultSet;
public class SingleExample {
public static void main(String[] args) throws IOException {
// If connect failed will throw a exception.
HugeClient hugeClient = HugeClient.builder("http://localhost:8080",
"hugegraph")
.build();
SchemaManager schema = hugeClient.schema();
schema.propertyKey("name").asText().ifNotExist().create();
schema.propertyKey("age").asInt().ifNotExist().create();
schema.propertyKey("city").asText().ifNotExist().create();
schema.propertyKey("weight").asDouble().ifNotExist().create();
schema.propertyKey("lang").asText().ifNotExist().create();
schema.propertyKey("date").asDate().ifNotExist().create();
schema.propertyKey("price").asInt().ifNotExist().create();
schema.vertexLabel("person")
.properties("name", "age", "city")
.primaryKeys("name")
.ifNotExist()
.create();
schema.vertexLabel("software")
.properties("name", "lang", "price")
.primaryKeys("name")
.ifNotExist()
.create();
schema.indexLabel("personByCity")
.onV("person")
.by("city")
.secondary()
.ifNotExist()
.create();
schema.indexLabel("personByAgeAndCity")
.onV("person")
.by("age", "city")
.secondary()
.ifNotExist()
.create();
schema.indexLabel("softwareByPrice")
.onV("software")
.by("price")
.range()
.ifNotExist()
.create();
schema.edgeLabel("knows")
.sourceLabel("person")
.targetLabel("person")
.properties("date", "weight")
.ifNotExist()
.create();
schema.edgeLabel("created")
.sourceLabel("person").targetLabel("software")
.properties("date", "weight")
.ifNotExist()
.create();
schema.indexLabel("createdByDate")
.onE("created")
.by("date")
.secondary()
.ifNotExist()
.create();
schema.indexLabel("createdByWeight")
.onE("created")
.by("weight")
.range()
.ifNotExist()
.create();
schema.indexLabel("knowsByWeight")
.onE("knows")
.by("weight")
.range()
.ifNotExist()
.create();
GraphManager graph = hugeClient.graph();
Vertex marko = graph.addVertex(T.LABEL, "person", "name", "marko",
"age", 29, "city", "Beijing");
Vertex vadas = graph.addVertex(T.LABEL, "person", "name", "vadas",
"age", 27, "city", "Hongkong");
Vertex lop = graph.addVertex(T.LABEL, "software", "name", "lop",
"lang", "java", "price", 328);
Vertex josh = graph.addVertex(T.LABEL, "person", "name", "josh",
"age", 32, "city", "Beijing");
Vertex ripple = graph.addVertex(T.LABEL, "software", "name", "ripple",
"lang", "java", "price", 199);
Vertex peter = graph.addVertex(T.LABEL, "person", "name", "peter",
"age", 35, "city", "Shanghai");
marko.addEdge("knows", vadas, "date", "2016-01-10", "weight", 0.5);
marko.addEdge("knows", josh, "date", "2013-02-20", "weight", 1.0);
marko.addEdge("created", lop, "date", "2017-12-10", "weight", 0.4);
josh.addEdge("created", lop, "date", "2009-11-11", "weight", 0.4);
josh.addEdge("created", ripple, "date", "2017-12-10", "weight", 1.0);
peter.addEdge("created", lop, "date", "2017-03-24", "weight", 0.2);
GremlinManager gremlin = hugeClient.gremlin();
System.out.println("==== Path ====");
ResultSet resultSet = gremlin.gremlin("g.V().outE().path()").execute();
Iterator<Result> results = resultSet.iterator();
results.forEachRemaining(result -> {
System.out.println(result.getObject().getClass());
Object object = result.getObject();
if (object instanceof Vertex) {
System.out.println(((Vertex) object).id());
} else if (object instanceof Edge) {
System.out.println(((Edge) object).id());
} else if (object instanceof Path) {
List<Object> elements = ((Path) object).objects();
elements.forEach(element -> {
System.out.println(element.getClass());
System.out.println(element);
});
} else {
System.out.println(object);
}
});
hugeClient.close();
}
}
4.3.2 BatchExample
import java.util.ArrayList;
import java.util.List;
import org.apache.hugegraph.driver.GraphManager;
import org.apache.hugegraph.driver.HugeClient;
import org.apache.hugegraph.driver.SchemaManager;
import org.apache.hugegraph.structure.graph.Edge;
import org.apache.hugegraph.structure.graph.Vertex;
public class BatchExample {
public static void main(String[] args) {
// If connect failed will throw a exception.
HugeClient hugeClient = HugeClient.builder("http://localhost:8080",
"hugegraph")
.build();
SchemaManager schema = hugeClient.schema();
schema.propertyKey("name").asText().ifNotExist().create();
schema.propertyKey("age").asInt().ifNotExist().create();
schema.propertyKey("lang").asText().ifNotExist().create();
schema.propertyKey("date").asDate().ifNotExist().create();
schema.propertyKey("price").asInt().ifNotExist().create();
schema.vertexLabel("person")
.properties("name", "age")
.primaryKeys("name")
.ifNotExist()
.create();
schema.vertexLabel("person")
.properties("price")
.nullableKeys("price")
.append();
schema.vertexLabel("software")
.properties("name", "lang", "price")
.primaryKeys("name")
.ifNotExist()
.create();
schema.indexLabel("softwareByPrice")
.onV("software").by("price")
.range()
.ifNotExist()
.create();
schema.edgeLabel("knows")
.link("person", "person")
.properties("date")
.ifNotExist()
.create();
schema.edgeLabel("created")
.link("person", "software")
.properties("date")
.ifNotExist()
.create();
schema.indexLabel("createdByDate")
.onE("created").by("date")
.secondary()
.ifNotExist()
.create();
// get schema object by name
System.out.println(schema.getPropertyKey("name"));
System.out.println(schema.getVertexLabel("person"));
System.out.println(schema.getEdgeLabel("knows"));
System.out.println(schema.getIndexLabel("createdByDate"));
// list all schema objects
System.out.println(schema.getPropertyKeys());
System.out.println(schema.getVertexLabels());
System.out.println(schema.getEdgeLabels());
System.out.println(schema.getIndexLabels());
GraphManager graph = hugeClient.graph();
Vertex marko = new Vertex("person").property("name", "marko")
.property("age", 29);
Vertex vadas = new Vertex("person").property("name", "vadas")
.property("age", 27);
Vertex lop = new Vertex("software").property("name", "lop")
.property("lang", "java")
.property("price", 328);
Vertex josh = new Vertex("person").property("name", "josh")
.property("age", 32);
Vertex ripple = new Vertex("software").property("name", "ripple")
.property("lang", "java")
.property("price", 199);
Vertex peter = new Vertex("person").property("name", "peter")
.property("age", 35);
Edge markoKnowsVadas = new Edge("knows").source(marko).target(vadas)
.property("date", "2016-01-10");
Edge markoKnowsJosh = new Edge("knows").source(marko).target(josh)
.property("date", "2013-02-20");
Edge markoCreateLop = new Edge("created").source(marko).target(lop)
.property("date",
"2017-12-10");
Edge joshCreateRipple = new Edge("created").source(josh).target(ripple)
.property("date",
"2017-12-10");
Edge joshCreateLop = new Edge("created").source(josh).target(lop)
.property("date", "2009-11-11");
Edge peterCreateLop = new Edge("created").source(peter).target(lop)
.property("date",
"2017-03-24");
List<Vertex> vertices = new ArrayList<>();
vertices.add(marko);
vertices.add(vadas);
vertices.add(lop);
vertices.add(josh);
vertices.add(ripple);
vertices.add(peter);
List<Edge> edges = new ArrayList<>();
edges.add(markoKnowsVadas);
edges.add(markoKnowsJosh);
edges.add(markoCreateLop);
edges.add(joshCreateRipple);
edges.add(joshCreateLop);
edges.add(peterCreateLop);
vertices = graph.addVertices(vertices);
vertices.forEach(vertex -> System.out.println(vertex));
edges = graph.addEdges(edges, false);
edges.forEach(edge -> System.out.println(edge));
hugeClient.close();
}
}
4.4 运行 Example
运行 Example 之前需要启动 Server, 启动过程见HugeGraph-Server Quick Start
4.5 详细 API 说明
6 - HugeGraph-Tools Quick Start
1 HugeGraph-Tools概述
HugeGraph-Tools 是 HugeGraph 的自动化部署、管理和备份/还原组件。
2 获取 HugeGraph-Tools
有两种方式可以获取 HugeGraph-Tools:(它被包含子 Toolchain 中)
- 下载二进制tar包
- 下载源码编译安装
2.1 下载二进制tar包
下载最新版本的 HugeGraph-Toolchain 包, 然后进入 tools 子目录
wget https://downloads.apache.org/incubator/hugegraph/1.0.0/apache-hugegraph-toolchain-incubating-1.0.0.tar.gz
tar zxf *hugegraph*.tar.gz
2.2 下载源码编译安装
源码编译前请确保安装了wget命令
下载最新版本的 HugeGraph-Toolchain 源码包, 然后根目录编译或者单独编译 tool 子模块:
# 1. get from github
git clone https://github.com/apache/hugegraph-toolchain.git
# 2. get from direct (e.g. here is 1.0.0, please choose the latest version)
wget https://downloads.apache.org/incubator/hugegraph/1.0.0/apache-hugegraph-toolchain-incubating-1.0.0-src.tar.gz
编译生成 tar 包:
cd hugegraph-tools
mvn package -DskipTests
生成 tar 包 hugegraph-tools-${version}.tar.gz
3 使用
3.1 功能概览
解压后,进入 hugegraph-tools 目录,可以使用bin/hugegraph
或者bin/hugegraph help
来查看 usage 信息。主要分为:
- 图管理类,graph-mode-set、graph-mode-get、graph-list、graph-get 和 graph-clear
- 异步任务管理类,task-list、task-get、task-delete、task-cancel 和 task-clear
- Gremlin类,gremlin-execute 和 gremlin-schedule
- 备份/恢复类,backup、restore、migrate、schedule-backup 和 dump
- 安装部署类,deploy、clear、start-all 和 stop-all
Usage: hugegraph [options] [command] [command options]
3.2 [options]-全局变量
options
是 HugeGraph-Tools 的全局变量,可以在 hugegraph-tools/bin/hugegraph 中配置,包括:
- –graph,HugeGraph-Tools 操作的图的名字,默认值是 hugegraph
- –url,HugeGraph-Server 的服务地址,默认是 http://127.0.0.1:8080
- –user,当 HugeGraph-Server 开启认证时,传递用户名
- –password,当 HugeGraph-Server 开启认证时,传递用户的密码
- –timeout,连接 HugeGraph-Server 时的超时时间,默认是 30s
- –trust-store-file,证书文件的路径,当 –url 使用 https 时,HugeGraph-Client 使用的 truststore 文件,默认为空,代表使用 hugegraph-tools 内置的 truststore 文件 conf/hugegraph.truststore
- –trust-store-password,证书文件的密码,当 –url 使用 https 时,HugeGraph-Client 使用的 truststore 的密码,默认为空,代表使用 hugegraph-tools 内置的 truststore 文件的密码
上述全局变量,也可以通过环境变量来设置。一种方式是在命令行使用 export 设置临时环境变量,在该命令行关闭之前均有效
全局变量 | 环境变量 | 示例 |
---|---|---|
–url | HUGEGRAPH_URL | export HUGEGRAPH_URL=http://127.0.0.1:8080 |
–graph | HUGEGRAPH_GRAPH | export HUGEGRAPH_GRAPH=hugegraph |
–user | HUGEGRAPH_USERNAME | export HUGEGRAPH_USERNAME=admin |
–password | HUGEGRAPH_PASSWORD | export HUGEGRAPH_PASSWORD=test |
–timeout | HUGEGRAPH_TIMEOUT | export HUGEGRAPH_TIMEOUT=30 |
–trust-store-file | HUGEGRAPH_TRUST_STORE_FILE | export HUGEGRAPH_TRUST_STORE_FILE=/tmp/trust-store |
–trust-store-password | HUGEGRAPH_TRUST_STORE_PASSWORD | export HUGEGRAPH_TRUST_STORE_PASSWORD=xxxx |
另一种方式是在 bin/hugegraph 脚本中设置环境变量:
#!/bin/bash
# Set environment here if needed
#export HUGEGRAPH_URL=
#export HUGEGRAPH_GRAPH=
#export HUGEGRAPH_USERNAME=
#export HUGEGRAPH_PASSWORD=
#export HUGEGRAPH_TIMEOUT=
#export HUGEGRAPH_TRUST_STORE_FILE=
#export HUGEGRAPH_TRUST_STORE_PASSWORD=
3.3 图管理类,graph-mode-set、graph-mode-get、graph-list、graph-get和graph-clear
- graph-mode-set,设置图的 restore mode
- –graph-mode 或者 -m,必填项,指定将要设置的模式,合法值包括 [NONE, RESTORING, MERGING, LOADING]
- graph-mode-get,获取图的 restore mode
- graph-list,列出某个 HugeGraph-Server 中全部的图
- graph-get,获取某个图及其存储后端类型
- graph-clear,清除某个图的全部 schema 和 data
- –confirm-message 或者 -c,必填项,删除确认信息,需要手动输入,二次确认防止误删,“I’m sure to delete all data”,包括双引号
当需要把备份的图原样恢复到一个新的图中的时候,需要先将图模式设置为 RESTORING 模式;当需要将备份的图合并到已存在的图中时,需要先将图模式设置为 MERGING 模式。
3.4 异步任务管理类,task-list、task-get和task-delete
- task-list,列出某个图中的异步任务,可以根据任务的状态过滤
- –status,选填项,指定要查看的任务的状态,即按状态过滤任务
- –limit,选填项,指定要获取的任务的数目,默认为 -1,意思为获取全部符合条件的任务
- task-get,获取某个异步任务的详细信息
- –task-id,必填项,指定异步任务的 ID
- task-delete,删除某个异步任务的信息
- –task-id,必填项,指定异步任务的 ID
- task-cancel,取消某个异步任务的执行
- –task-id,要取消的异步任务的 ID
- task-clear,清理完成的异步任务
- –force,选填项,设置时,表示清理全部异步任务,未执行完成的先取消,然后清除所有异步任务。默认只清理已完成的异步任务
3.5 Gremlin类,gremlin-execute和gremlin-schedule
- gremlin-execute,发送 Gremlin 语句到 HugeGraph-Server 来执行查询或修改操作,同步执行,结束后返回结果
- –file 或者 -f,指定要执行的脚本文件,UTF-8编码,与 –script 互斥
- –script 或者 -s,指定要执行的脚本字符串,与 –file 互斥
- –aliases 或者 -a,Gremlin 别名设置,格式为:key1=value1,key2=value2,…
- –bindings 或者 -b,Gremlin 绑定设置,格式为:key1=value1,key2=value2,…
- –language 或者 -l,Gremlin 脚本的语言,默认为 gremlin-groovy
–file 和 –script 二者互斥,必须设置其中之一
- gremlin-schedule,发送 Gremlin 语句到 HugeGraph-Server 来执行查询或修改操作,异步执行,任务提交后立刻返回异步任务id
- –file 或者 -f,指定要执行的脚本文件,UTF-8编码,与 –script 互斥
- –script 或者 -s,指定要执行的脚本字符串,与 –file 互斥
- –bindings 或者 -b,Gremlin 绑定设置,格式为:key1=value1,key2=value2,…
- –language 或者 -l,Gremlin 脚本的语言,默认为 gremlin-groovy
–file 和 –script 二者互斥,必须设置其中之一
3.6 备份/恢复类
- backup,将某张图中的 schema 或者 data 备份到 HugeGraph 系统之外,以 JSON 形式存在本地磁盘或者 HDFS
- –format,备份的格式,可选值包括 [json, text],默认为 json
- –all-properties,是否备份顶点/边全部的属性,仅在 –format 为 text 是有效,默认 false
- –label,要备份的顶点/边的类型,仅在 –format 为 text 是有效,只有备份顶点或者边的时候有效
- –properties,要备份的顶点/边的属性,逗号分隔,仅在 –format 为 text 是有效,只有备份顶点或者边的时候有效
- –compress,备份时是否压缩数据,默认为 true
- –directory 或者 -d,存储 schema 或者 data 的目录,本地目录时,默认为’./{graphName}’,HDFS 时,默认为 ‘{fs.default.name}/{graphName}’
- –huge-types 或者 -t,要备份的数据类型,逗号分隔,可选值为 ‘all’ 或者 一个或多个 [vertex,edge,vertex_label,edge_label,property_key,index_label] 的组合,‘all’ 代表全部6种类型,即顶点、边和所有schema
- –log 或者 -l,指定日志目录,默认为当前目录
- –retry,指定失败重试次数,默认为 3
- –split-size 或者 -s,指定在备份时对顶点或者边分块的大小,默认为 1048576
- -D,用 -Dkey=value 的模式指定动态参数,用来备份数据到 HDFS 时,指定 HDFS 的配置项,例如:-Dfs.default.name=hdfs://localhost:9000
- restore,将 JSON 格式存储的 schema 或者 data 恢复到一个新图中(RESTORING 模式)或者合并到已存在的图中(MERGING 模式)
- –directory 或者 -d,存储 schema 或者 data 的目录,本地目录时,默认为’./{graphName}’,HDFS 时,默认为 ‘{fs.default.name}/{graphName}’
- –clean,是否在恢复图完成后删除 –directory 指定的目录,默认为 false
- –huge-types 或者 -t,要恢复的数据类型,逗号分隔,可选值为 ‘all’ 或者 一个或多个 [vertex,edge,vertex_label,edge_label,property_key,index_label] 的组合,‘all’ 代表全部6种类型,即顶点、边和所有schema
- –log 或者 -l,指定日志目录,默认为当前目录
- –retry,指定失败重试次数,默认为 3
- -D,用 -Dkey=value 的模式指定动态参数,用来从 HDFS 恢复图时,指定 HDFS 的配置项,例如:-Dfs.default.name=hdfs://localhost:9000
只有当 –format 为 json 执行 backup 时,才可以使用 restore 命令恢复
- migrate, 将当前连接的图迁移至另一个 HugeGraphServer 中
- –target-graph,目标图的名字,默认为 hugegraph
- –target-url,目标图所在的 HugeGraphServer,默认为 http://127.0.0.1:8081
- –target-username,访问目标图的用户名
- –target-password,访问目标图的密码
- –target-timeout,访问目标图的超时时间
- –target-trust-store-file,访问目标图使用的 truststore 文件
- –target-trust-store-password,访问目标图使用的 truststore 的密码
- –directory 或者 -d,迁移过程中,存储源图的 schema 或者 data 的目录,本地目录时,默认为’./{graphName}’,HDFS 时,默认为 ‘{fs.default.name}/{graphName}’
- –huge-types 或者 -t,要迁移的数据类型,逗号分隔,可选值为 ‘all’ 或者 一个或多个 [vertex,edge,vertex_label,edge_label,property_key,index_label] 的组合,‘all’ 代表全部6种类型,即顶点、边和所有schema
- –log 或者 -l,指定日志目录,默认为当前目录
- –retry,指定失败重试次数,默认为 3
- –split-size 或者 -s,指定迁移过程中对源图进行备份时顶点或者边分块的大小,默认为 1048576
- -D,用 -Dkey=value 的模式指定动态参数,用来在迁移图过程中需要备份数据到 HDFS 时,指定 HDFS 的配置项,例如:-Dfs.default.name=hdfs://localhost:9000
- –graph-mode 或者 -m,将源图恢复到目标图时将目标图设置的模式,合法值包括 [RESTORING, MERGING]
- –keep-local-data,是否保留在迁移图的过程中产生的源图的备份,默认为 false,即默认迁移图结束后不保留产生的源图备份
- schedule-backup,周期性对图执行备份操作,并保留一定数目的最新备份(目前仅支持本地文件系统)
- –directory 或者 -d,必填项,指定备份数据的目录
- –backup-num,选填项,指定保存的最新的备份的数目,默认为 3
- –interval,选填项,指定进行备份的周期,格式同 Linux crontab 格式
- dump,把整张图的顶点和边全部导出,默认以
vertex vertex-edge1 vertex-edge2...
JSON格式存储。 用户也可以自定义存储格式,只需要在hugegraph-tools/src/main/java/com/baidu/hugegraph/formatter
目录下实现一个继承自Formatter
的类,例如CustomFormatter
,使用时指定该类为formatter即可,例如bin/hugegraph dump -f CustomFormatter
- –formatter 或者 -f,指定使用的 formatter,默认为 JsonFormatter
- –directory 或者 -d,存储 schema 或者 data 的目录,默认为当前目录
- –log 或者 -l,指定日志目录,默认为当前目录
- –retry,指定失败重试次数,默认为 3
- –split-size 或者 -s,指定在备份时对顶点或者边分块的大小,默认为 1048576
- -D,用 -Dkey=value 的模式指定动态参数,用来备份数据到 HDFS 时,指定 HDFS 的配置项,例如:-Dfs.default.name=hdfs://localhost:9000
3.7 安装部署类
- deploy,一键下载、安装和启动 HugeGraph-Server 和 HugeGraph-Studio
- -v,必填项,指明安装的 HugeGraph-Server 和 HugeGraph-Studio 的版本号,最新的是 0.9
- -p,必填项,指定安装的 HugeGraph-Server 和 HugeGraph-Studio 目录
- -u,选填项,指定下载 HugeGraph-Server 和 HugeGraph-Studio 压缩包的链接
- clear,清理 HugeGraph-Server 和 HugeGraph-Studio 目录和tar包
- -p,必填项,指定要清理的 HugeGraph-Server 和 HugeGraph-Studio 的目录
- start-all,一键启动 HugeGraph-Server 和 HugeGraph-Studio,并启动监控,服务死掉时自动拉起服务
- -v,必填项,指明要启动的 HugeGraph-Server 和 HugeGraph-Studio 的版本号,最新的是 0.9
- -p,必填项,指定安装了 HugeGraph-Server 和 HugeGraph-Studio 的目录
- stop-all,一键关闭 HugeGraph-Server 和 HugeGraph-Studio
deploy命令中有可选参数 -u,提供时会使用指定的下载地址替代默认下载地址下载 tar 包,并且将地址写入
~/hugegraph-download-url-prefix
文件中;之后如果不指定地址时,会优先从~/hugegraph-download-url-prefix
指定的地址下载 tar 包;如果 -u 和~/hugegraph-download-url-prefix
都没有时,会从默认下载地址进行下载
3.8 具体命令参数
各子命令的具体参数如下:
Usage: hugegraph [options] [command] [command options]
Options:
--graph
Name of graph
Default: hugegraph
--password
Password of user
--timeout
Connection timeout
Default: 30
--trust-store-file
The path of client truststore file used when https protocol is enabled
--trust-store-password
The password of the client truststore file used when the https protocol
is enabled
--url
The URL of HugeGraph-Server
Default: http://127.0.0.1:8080
--user
Name of user
Commands:
graph-list List all graphs
Usage: graph-list
graph-get Get graph info
Usage: graph-get
graph-clear Clear graph schema and data
Usage: graph-clear [options]
Options:
* --confirm-message, -c
Confirm message of graph clear is "I'm sure to delete all data".
(Note: include "")
graph-mode-set Set graph mode
Usage: graph-mode-set [options]
Options:
* --graph-mode, -m
Graph mode, include: [NONE, RESTORING, MERGING]
Possible Values: [NONE, RESTORING, MERGING, LOADING]
graph-mode-get Get graph mode
Usage: graph-mode-get
task-list List tasks
Usage: task-list [options]
Options:
--limit
Limit number, no limit if not provided
Default: -1
--status
Status of task
task-get Get task info
Usage: task-get [options]
Options:
* --task-id
Task id
Default: 0
task-delete Delete task
Usage: task-delete [options]
Options:
* --task-id
Task id
Default: 0
task-cancel Cancel task
Usage: task-cancel [options]
Options:
* --task-id
Task id
Default: 0
task-clear Clear completed tasks
Usage: task-clear [options]
Options:
--force
Force to clear all tasks, cancel all uncompleted tasks firstly,
and delete all completed tasks
Default: false
gremlin-execute Execute Gremlin statements
Usage: gremlin-execute [options]
Options:
--aliases, -a
Gremlin aliases, valid format is: 'key1=value1,key2=value2...'
Default: {}
--bindings, -b
Gremlin bindings, valid format is: 'key1=value1,key2=value2...'
Default: {}
--file, -f
Gremlin Script file to be executed, UTF-8 encoded, exclusive to
--script
--language, -l
Gremlin script language
Default: gremlin-groovy
--script, -s
Gremlin script to be executed, exclusive to --file
gremlin-schedule Execute Gremlin statements as asynchronous job
Usage: gremlin-schedule [options]
Options:
--bindings, -b
Gremlin bindings, valid format is: 'key1=value1,key2=value2...'
Default: {}
--file, -f
Gremlin Script file to be executed, UTF-8 encoded, exclusive to
--script
--language, -l
Gremlin script language
Default: gremlin-groovy
--script, -s
Gremlin script to be executed, exclusive to --file
backup Backup graph schema/data. If directory is on HDFS, use -D to
set HDFS params. For exmaple:
-Dfs.default.name=hdfs://localhost:9000
Usage: backup [options]
Options:
--all-properties
All properties to be backup flag
Default: false
--compress
compress flag
Default: true
--directory, -d
Directory of graph schema/data, default is './{graphname}' in
local file system or '{fs.default.name}/{graphname}' in HDFS
--format
File format, valid is [json, text]
Default: json
--huge-types, -t
Type of schema/data. Concat with ',' if more than one. 'all' means
all vertices, edges and schema, in other words, 'all' equals with
'vertex,edge,vertex_label,edge_label,property_key,index_label'
Default: [PROPERTY_KEY, VERTEX_LABEL, EDGE_LABEL, INDEX_LABEL, VERTEX, EDGE]
--label
Vertex or edge label, only valid when type is vertex or edge
--log, -l
Directory of log
Default: ./logs
--properties
Vertex or edge properties to backup, only valid when type is
vertex or edge
Default: []
--retry
Retry times, default is 3
Default: 3
--split-size, -s
Split size of shard
Default: 1048576
-D
HDFS config parameters
Syntax: -Dkey=value
Default: {}
schedule-backup Schedule backup task
Usage: schedule-backup [options]
Options:
--backup-num
The number of latest backups to keep
Default: 3
* --directory, -d
The directory of backups stored
--interval
The interval of backup, format is: "a b c d e". 'a' means minute
(0 - 59), 'b' means hour (0 - 23), 'c' means day of month (1 -
31), 'd' means month (1 - 12), 'e' means day of week (0 - 6)
(Sunday=0), "*" means all
Default: "0 0 * * *"
dump Dump graph to files
Usage: dump [options]
Options:
--directory, -d
Directory of graph schema/data, default is './{graphname}' in
local file system or '{fs.default.name}/{graphname}' in HDFS
--formatter, -f
Formatter to customize format of vertex/edge
Default: JsonFormatter
--log, -l
Directory of log
Default: ./logs
--retry
Retry times, default is 3
Default: 3
--split-size, -s
Split size of shard
Default: 1048576
-D
HDFS config parameters
Syntax: -Dkey=value
Default: {}
restore Restore graph schema/data. If directory is on HDFS, use -D to
set HDFS params if needed. For
exmaple:-Dfs.default.name=hdfs://localhost:9000
Usage: restore [options]
Options:
--clean
Whether to remove the directory of graph data after restored
Default: false
--directory, -d
Directory of graph schema/data, default is './{graphname}' in
local file system or '{fs.default.name}/{graphname}' in HDFS
--huge-types, -t
Type of schema/data. Concat with ',' if more than one. 'all' means
all vertices, edges and schema, in other words, 'all' equals with
'vertex,edge,vertex_label,edge_label,property_key,index_label'
Default: [PROPERTY_KEY, VERTEX_LABEL, EDGE_LABEL, INDEX_LABEL, VERTEX, EDGE]
--log, -l
Directory of log
Default: ./logs
--retry
Retry times, default is 3
Default: 3
-D
HDFS config parameters
Syntax: -Dkey=value
Default: {}
migrate Migrate graph
Usage: migrate [options]
Options:
--directory, -d
Directory of graph schema/data, default is './{graphname}' in
local file system or '{fs.default.name}/{graphname}' in HDFS
--graph-mode, -m
Mode used when migrating to target graph, include: [RESTORING,
MERGING]
Default: RESTORING
Possible Values: [NONE, RESTORING, MERGING, LOADING]
--huge-types, -t
Type of schema/data. Concat with ',' if more than one. 'all' means
all vertices, edges and schema, in other words, 'all' equals with
'vertex,edge,vertex_label,edge_label,property_key,index_label'
Default: [PROPERTY_KEY, VERTEX_LABEL, EDGE_LABEL, INDEX_LABEL, VERTEX, EDGE]
--keep-local-data
Whether to keep the local directory of graph data after restored
Default: false
--log, -l
Directory of log
Default: ./logs
--retry
Retry times, default is 3
Default: 3
--split-size, -s
Split size of shard
Default: 1048576
--target-graph
The name of target graph to migrate
Default: hugegraph
--target-password
The password of target graph to migrate
--target-timeout
The timeout to connect target graph to migrate
Default: 0
--target-trust-store-file
The trust store file of target graph to migrate
--target-trust-store-password
The trust store password of target graph to migrate
--target-url
The url of target graph to migrate
Default: http://127.0.0.1:8081
--target-user
The username of target graph to migrate
-D
HDFS config parameters
Syntax: -Dkey=value
Default: {}
deploy Install HugeGraph-Server and HugeGraph-Studio
Usage: deploy [options]
Options:
* -p
Install path of HugeGraph-Server and HugeGraph-Studio
-u
Download url prefix path of HugeGraph-Server and HugeGraph-Studio
* -v
Version of HugeGraph-Server and HugeGraph-Studio
start-all Start HugeGraph-Server and HugeGraph-Studio
Usage: start-all [options]
Options:
* -p
Install path of HugeGraph-Server and HugeGraph-Studio
* -v
Version of HugeGraph-Server and HugeGraph-Studio
clear Clear HugeGraph-Server and HugeGraph-Studio
Usage: clear [options]
Options:
* -p
Install path of HugeGraph-Server and HugeGraph-Studio
stop-all Stop HugeGraph-Server and HugeGraph-Studio
Usage: stop-all
help Print usage
Usage: help
3.9 具体命令示例
1. gremlin语句
# 同步执行gremlin
./bin/hugegraph --url http://127.0.0.1:8080 --graph hugegraph gremlin-execute --script 'g.V().count()'
# 异步执行gremlin
./bin/hugegraph --url http://127.0.0.1:8080 --graph hugegraph gremlin-schedule --script 'g.V().count()'
2. 查看task情况
./bin/hugegraph --url http://127.0.0.1:8080 --graph hugegraph task-list
./bin/hugegraph --url http://127.0.0.1:8080 --graph hugegraph task-list --limit 5
./bin/hugegraph --url http://127.0.0.1:8080 --graph hugegraph task-list --status success
3. 图模式查看和设置
./bin/hugegraph --url http://127.0.0.1:8080 --graph hugegraph graph-mode-set -m RESTORING MERGING NONE
./bin/hugegraph --url http://127.0.0.1:8080 --graph hugegraph graph-mode-set -m RESTORING
./bin/hugegraph --url http://127.0.0.1:8080 --graph hugegraph graph-mode-get
./bin/hugegraph --url http://127.0.0.1:8080 --graph hugegraph graph-list
4. 清理图
./bin/hugegraph --url http://127.0.0.1:8080 --graph hugegraph graph-clear -c "I'm sure to delete all data"
5. 图备份
./bin/hugegraph --url http://127.0.0.1:8080 --graph hugegraph backup -t all --directory ./backup-test
6. 周期性的备份
./bin/hugegraph --url http://127.0.0.1:8080 --graph hugegraph --interval */2 * * * * schedule-backup -d ./backup-0.10.2
7. 图恢复
# 设置图模式
./bin/hugegraph --url http://127.0.0.1:8080 --graph hugegraph graph-mode-set -m RESTORING
# 恢复图
./bin/hugegraph --url http://127.0.0.1:8080 --graph hugegraph restore -t all --directory ./backup-test
# 恢复图模式
./bin/hugegraph --url http://127.0.0.1:8080 --graph hugegraph graph-mode-set -m NONE
8. 图迁移
./bin/hugegraph --url http://127.0.0.1:8080 --graph hugegraph migrate --target-url http://127.0.0.1:8090 --target-graph hugegraph
7 - HugeGraph-Computer Quick Start
1 HugeGraph-Computer 概述
HugeGraph-Computer
是分布式图处理系统 (OLAP). 它是 Pregel 的一个实现. 它可以运行在 Kubernetes 上。
特性
- 支持分布式MPP图计算,集成HugeGraph作为图输入输出存储。
- 算法基于BSP(Bulk Synchronous Parallel)模型,通过多次并行迭代进行计算,每一次迭代都是一次超步。
- 自动内存管理。该框架永远不会出现 OOM(内存不足),因为如果它没有足够的内存来容纳所有数据,它会将一些数据拆分到磁盘。
- 边的部分或超级节点的消息可以在内存中,所以你永远不会丢失它。
- 您可以从 HDFS 或 HugeGraph 或任何其他系统加载数据。
- 您可以将结果输出到 HDFS 或 HugeGraph,或任何其他系统。
- 易于开发新算法。您只需要像在单个服务器中一样专注于仅顶点处理,而不必担心消息传输和内存存储管理。
2 依赖
2.1 安装 Java 11 (JDK 11)
必须在 ≥ Java 11
的环境上启动 Computer
,然后自行配置。
在往下阅读之前务必执行 java -version
命令查看 jdk 版本
3 开始
3.1 在本地运行 PageRank 算法
要使用 HugeGraph-Computer 运行算法,必须装有 Java 11 或更高版本。
还需要首先部署 HugeGraph-Server 和 Etcd.
有两种方式可以获取 HugeGraph-Computer:
- 下载已编译的压缩包
- 克隆源码编译打包
3.1.1 下载已编译的压缩包
下载最新版本的 HugeGraph-Computer release 包:
wget https://downloads.apache.org/incubator/hugegraph/${version}/apache-hugegraph-computer-incubating-${version}.tar.gz
tar zxvf apache-hugegraph-computer-incubating-${version}.tar.gz -C hugegraph-computer
3.1.2 克隆源码编译打包
克隆最新版本的 HugeGraph-Computer 源码包:
$ git clone https://github.com/apache/hugegraph-computer.git
编译生成tar包:
cd hugegraph-computer
mvn clean package -DskipTests
3.1.3 启动 master 节点
您可以使用
-c
参数指定配置文件, 更多computer 配置请看: Computer Config Options
cd hugegraph-computer
bin/start-computer.sh -d local -r master
3.1.4 启动 worker 节点
bin/start-computer.sh -d local -r worker
3.1.5 查询算法结果
2.5.1 为 server 启用 OLAP
索引查询
如果没有启用OLAP索引,则需要启用, 更多参考: modify-graphs-read-mode
PUT http://localhost:8080/graphs/hugegraph/graph_read_mode
"ALL"
3.1.5.2 查询 page_rank
属性值:
curl "http://localhost:8080/graphs/hugegraph/graph/vertices?page&limit=3" | gunzip
3.2 在 Kubernetes 中运行 PageRank 算法
要使用 HugeGraph-Computer 运行算法,您需要先部署 HugeGraph-Server
3.2.1 安装 HugeGraph-Computer CRD
# Kubernetes version >= v1.16
kubectl apply -f https://raw.githubusercontent.com/apache/hugegraph-computer/master/computer-k8s-operator/manifest/hugegraph-computer-crd.v1.yaml
# Kubernetes version < v1.16
kubectl apply -f https://raw.githubusercontent.com/apache/hugegraph-computer/master/computer-k8s-operator/manifest/hugegraph-computer-crd.v1beta1.yaml
3.2.2 显示 CRD
kubectl get crd
NAME CREATED AT
hugegraphcomputerjobs.hugegraph.apache.org 2021-09-16T08:01:08Z
3.2.3 安装 hugegraph-computer-operator&etcd-server
kubectl apply -f https://raw.githubusercontent.com/apache/hugegraph-computer/master/computer-k8s-operator/manifest/hugegraph-computer-operator.yaml
3.2.4 等待 hugegraph-computer-operator&etcd-server 部署完成
kubectl get pod -n hugegraph-computer-operator-system
NAME READY STATUS RESTARTS AGE
hugegraph-computer-operator-controller-manager-58c5545949-jqvzl 1/1 Running 0 15h
hugegraph-computer-operator-etcd-28lm67jxk5 1/1 Running 0 15h
3.2.5 提交作业
更多 computer crd spec 请看: Computer CRD
更多 Computer 配置请看: Computer Config Options
cat <<EOF | kubectl apply --filename -
apiVersion: hugegraph.apache.org/v1
kind: HugeGraphComputerJob
metadata:
namespace: hugegraph-computer-operator-system
name: &jobName pagerank-sample
spec:
jobId: *jobName
algorithmName: page_rank
image: hugegraph/hugegraph-computer:latest # algorithm image url
jarFile: /hugegraph/hugegraph-computer/algorithm/builtin-algorithm.jar # algorithm jar path
pullPolicy: Always
workerCpu: "4"
workerMemory: "4Gi"
workerInstances: 5
computerConf:
job.partitions_count: "20"
algorithm.params_class: org.apache.hugegraph.computer.algorithm.centrality.pagerank.PageRankParams
hugegraph.url: http://${hugegraph-server-host}:${hugegraph-server-port} # hugegraph server url
hugegraph.name: hugegraph # hugegraph graph name
EOF
3.2.6 显示作业
kubectl get hcjob/pagerank-sample -n hugegraph-computer-operator-system
NAME JOBID JOBSTATUS
pagerank-sample pagerank-sample RUNNING
3.2.7 显示节点日志
# Show the master log
kubectl logs -l component=pagerank-sample-master -n hugegraph-computer-operator-system
# Show the worker log
kubectl logs -l component=pagerank-sample-worker -n hugegraph-computer-operator-system
# Show diagnostic log of a job
# 注意: 诊断日志仅在作业失败时存在,并且只会保存一小时。
kubectl get event --field-selector reason=ComputerJobFailed --field-selector involvedObject.name=pagerank-sample -n hugegraph-computer-operator-system
3.2.8 显示作业的成功事件
NOTE: it will only be saved for one hour
kubectl get event --field-selector reason=ComputerJobSucceed --field-selector involvedObject.name=pagerank-sample -n hugegraph-computer-operator-system
3.2.9 查询算法结果
如果输出到 Hugegraph-Server
则与 Locally 模式一致,如果输出到 HDFS
,请检查 hugegraph-computerresults{jobId}
目录下的结果文件。
4 内置算法文档
4.1 支持的算法列表:
中心性算法:
- PageRank
- BetweennessCentrality
- ClosenessCentrality
- DegreeCentrality
社区算法:
- ClusteringCoefficient
- Kcore
- Lpa
- TriangleCount
- Wcc
路径算法:
- RingsDetection
- RingsDetectionWithFilter
更多算法请看: Built-In algorithms
4.2 算法描述
TODO
5 算法开发指南
TODO
6 注意事项
- 如果computer-k8s模块下面的某些类不存在,你需要运行
mvn compile
来提前生成对应的类。
8 - HugeGraph-Vermeer Quick Start
一、Vermeer 概述
1.1 运行架构
Vermeer 是一个 Go
编写的高性能内存优先的图计算框架 (一次启动,任意执行),支持 15+ OLAP 图算法的极速计算 (大部分秒~分钟级别完成执行),包含 master 和 worker 两种角色。master 目前只有一个 (可增加 HA),worker 可以有多个。
master 是负责通信、转发、汇总的节点,计算量和占用资源量较少。worker 是计算节点,用于存储图数据和运行计算任务,占用大量内存和 cpu。grpc 和 rest 模块分别负责内部通信和外部调用。
该框架的运行配置可以通过命令行参数传入,也可以通过位于 config/
目录下的配置文件指定,--env
参数可以指定使用哪个配置文件,例如 --env=master
指定使用 master.ini
。需要注意 master 需要指定监听的端口号,worker 需要指定监听端口号和 master 的 ip:port
。
1.2 运行方法
在进入文件夹目录后输入 ./vermeer --env=master
或 ./vermeer --env=worker01
二、任务创建类 rest api
2.1 简介
此类 rest api 提供所有创建任务的功能,包括读取图数据和多种计算功能,提供异步返回和同步返回两种接口。返回的内容均包含所创建任务的信息。使用 vermeer 的整体流程是先创建读取图的任务,待图读取完毕后创建计算任务执行计算。图不会自动被删除,在一个图上运行多个计算任务无需多次重复读取,如需删除可用删除图接口。任务状态可分为读取任务状态和计算任务状态。通常情况下客户端仅需了解创建、任务中、任务结束和任务错误四种状态。图状态是图是否可用的判断依据,若图正在读取中或图状态错误,无法使用该图创建计算任务。图删除接口仅在 loaded 和 error 状态且该图无计算任务时可用。
可以使用的 url 如下:
- 异步返回接口 POST http://master_ip:port/tasks/create 仅返回任务创建是否成功,需通过主动查询任务状态判断是否完成。
- 同步返回接口 POST http://master_ip:port/tasks/create/sync 在任务结束后返回。
2.2 加载图数据
具体参数参考 Vermeer 参数列表文档。
request 示例:
POST http://localhost:8688/tasks/create
{
"task_type": "load",
"graph": "testdb",
"params": {
"load.parallel": "50",
"load.type": "local",
"load.vertex_files": "{\"localhost\":\"data/twitter-2010.v_[0,99]\"}",
"load.edge_files": "{\"localhost\":\"data/twitter-2010.e_[0,99]\"}",
"load.use_out_degree": "1",
"load.use_outedge": "1"
}
}
2.3 输出计算结果
所有的 vermeer 计算任务均支持多种结果输出方式,可自定义输出方式:local、hdfs、afs 或 hugegraph,在发送请求时的 params 参数下加入对应参数,即可生效。指定 output.need_statistics 为 1 时,支持计算结果统计信息输出,结果会写在接口任务信息内。统计模式算子目前支持 “count” 和 “modularity” 。但仅针对社区发现算法适用。
具体参数参考 Vermeer 参数列表文档。
request 示例:
POST http://localhost:8688/tasks/create
{
"task_type": "compute",
"graph": "testdb",
"params": {
"compute.algorithm": "pagerank",
"compute.parallel":"10",
"compute.max_step":"10",
"output.type":"local",
"output.parallel":"1",
"output.file_path":"result/pagerank"
}
}
三、支持的算法
3.1 PageRank
PageRank 算法又称网页排名算法,是一种由搜索引擎根据网页(节点)之间相互的超链接进行计算的技
术,用来体现网页(节点)的相关性和重要性。
- 如果一个网页被很多其他网页链接到,说明这个网页比较重要,也就是其 PageRank 值会相对较高。
- 如果一个 PageRank 值很高的网页链接到其他网页,那么被链接到的网页的 PageRank 值会相应地提高。
PageRank 算法适用于网页排序、社交网络重点人物发掘等场景。
request 示例:
POST http://localhost:8688/tasks/create
{
"task_type": "compute",
"graph": "testdb",
"params": {
"compute.algorithm": "pagerank",
"compute.parallel":"10",
"output.type":"local",
"output.parallel":"1",
"output.file_path":"result/pagerank",
"compute.max_step":"10"
}
}
3.2 WCC(弱连通分量)
弱连通分量,计算无向图中所有联通的子图,输出各顶点所属的弱联通子图 id,表明各个点之间的连通性,区分不同的连通社区。
request 示例:
POST http://localhost:8688/tasks/create
{
"task_type": "compute",
"graph": "testdb",
"params": {
"compute.algorithm": "wcc",
"compute.parallel":"10",
"output.type":"local",
"output.parallel":"1",
"output.file_path":"result/wcc",
"compute.max_step":"10"
}
}
3.3 LPA(标签传播)
标签传递算法,是一种图聚类算法,常用在社交网络中,用于发现潜在的社区。
request 示例:
POST http://localhost:8688/tasks/create
{
"task_type": "compute",
"graph": "testdb",
"params": {
"compute.algorithm": "lpa",
"compute.parallel":"10",
"output.type":"local",
"output.parallel":"1",
"output.file_path":"result/lpa",
"compute.max_step":"10"
}
}
3.4 Degree Centrality(度中心性)
度中心性算法,算法用于计算图中每个节点的度中心性值,支持无向图和有向图。度中心性是衡量节点重要性的重要指标,节点与其它节点的边越多,则节点的度中心性值越大,节点在图中的重要性也就越高。在无向图中,度中心性的计算是基于边信息统计节点出现次数,得出节点的度中心性的值,在有向图中则基于边的方向进行筛选,基于输入边或输出边信息统计节点出现次数,得到节点的入度值或出度值。它表明各个点的重要性,一般越重要的点度数越高。
request 示例:
POST http://localhost:8688/tasks/create
{
"task_type": "compute",
"graph": "testdb",
"params": {
"compute.algorithm": "degree",
"compute.parallel":"10",
"output.type":"local",
"output.parallel":"1",
"output.file_path":"result/degree",
"degree.direction":"both"
}
}
3.5 Closeness Centrality(紧密中心性)
紧密中心性(Closeness Centrality)用于计算一个节点到所有其他可达节点的最短距离的倒数,进行累积后归一化的值。紧密中心度可以用来衡量信息从该节点传输到其他节点的时间长短。节点的“Closeness Centrality”越大,其在所在图中的位置越靠近中心,适用于社交网络中关键节点发掘等场景。
request 示例:
POST http://localhost:8688/tasks/create
{
"task_type": "compute",
"graph": "testdb",
"params": {
"compute.algorithm": "closeness_centrality",
"compute.parallel":"10",
"output.type":"local",
"output.parallel":"1",
"output.file_path":"result/closeness_centrality",
"closeness_centrality.sample_rate":"0.01"
}
}
3.6 Betweenness Centrality(中介中心性算法)
中介中心性算法(Betweeness Centrality)判断一个节点具有"桥梁"节点的值,值越大说明它作为图中两点间必经路径的可能性越大,典型的例子包括社交网络中的共同关注的人。适用于衡量社群围绕某个节点的聚集程度。
request 示例:
POST http://localhost:8688/tasks/create
{
"task_type": "compute",
"graph": "testdb",
"params": {
"compute.algorithm": "betweenness_centrality",
"compute.parallel":"10",
"output.type":"local",
"output.parallel":"1",
"output.file_path":"result/betweenness_centrality",
"betweenness_centrality.sample_rate":"0.01"
}
}
3.7 Triangle Count(三角形计数)
三角形计数算法,用于计算通过每个顶点的三角形个数,适用于计算用户之间的关系,关联性是不是成三角形。三角形越多,代表图中节点关联程度越高,组织关系越严密。社交网络中的三角形表示存在有凝聚力的社区,识别三角形有助于理解网络中个人或群体的聚类和相互联系。在金融网络或交易网络中,三角形的存在可能表示存在可疑或欺诈活动,三角形计数可以帮助识别可能需要进一步调查的交易模式。
输出的结果为 每个顶点对应一个 Triangle Count,即为每个顶点所在三角形的个数。
注:该算法为无向图算法,忽略边的方向。
request 示例:
POST http://localhost:8688/tasks/create
{
"task_type": "compute",
"graph": "testdb",
"params": {
"compute.algorithm": "triangle_count",
"compute.parallel":"10",
"output.type":"local",
"output.parallel":"1",
"output.file_path":"result/triangle_count"
}
}
3.8 K-Core
K-Core 算法,标记所有度数为 K 的顶点,适用于图的剪枝,查找图的核心部分。
request 示例:
POST http://localhost:8688/tasks/create
{
"task_type": "compute",
"graph": "testdb",
"params": {
"compute.algorithm": "kcore",
"compute.parallel":"10",
"output.type":"local",
"output.parallel":"1",
"output.file_path":"result/kcore",
"kcore.degree_k":"5"
}
}
3.9 SSSP(单元最短路径)
单源最短路径算法,求一个点到其他所有点的最短距离。
request 示例:
POST http://localhost:8688/tasks/create
{
"task_type": "compute",
"graph": "testdb",
"params": {
"compute.algorithm": "sssp",
"compute.parallel":"10",
"output.type":"local",
"output.parallel":"1",
"output.file_path":"result/degree",
"sssp.source":"tom"
}
}
3.10 KOUT
以一个点为起点,获取这个点的 k 层的节点。
request 示例:
POST http://localhost:8688/tasks/create
{
"task_type": "compute",
"graph": "testdb",
"params": {
"compute.algorithm": "kout",
"compute.parallel":"10",
"output.type":"local",
"output.parallel":"1",
"output.file_path":"result/kout",
"kout.source":"tom",
"compute.max_step":"6"
}
}
3.11 Louvain
Louvain 算法是一种基于模块度的社区发现算法。其基本思想是网络中节点尝试遍历所有邻居的社区标签,并选择最大化模块度增量的社区标签。在最大化模块度之后,每个社区看成一个新的节点,重复直到模块度不再增大。
Vermeer 上实现的分布式 Louvain 算法受节点顺序、并行计算等因素影响,并且由于 Louvain 算法由于其遍历顺序的随机导致社区压缩也具有一定的随机性,导致重复多次执行可能存在不同的结果。但整体趋势不会有大的变化。
request 示例:
POST http://localhost:8688/tasks/create
{
"task_type": "compute",
"graph": "testdb",
"params": {
"compute.algorithm": "louvain",
"compute.parallel":"10",
"compute.max_step":"1000",
"louvain.threshold":"0.0000001",
"louvain.resolution":"1.0",
"louvain.step":"10",
"output.type":"local",
"output.parallel":"1",
"output.file_path":"result/louvain"
}
}
3.12 Jaccard 相似度系数
Jaccard index , 又称为 Jaccard 相似系数(Jaccard similarity coefficient)用于比较有限样本集之间的相似性与差异性。Jaccard 系数值越大,样本相似度越高。用于计算一个给定的源点,与图中其他所有点的 Jaccard 相似系数。
request 示例:
POST http://localhost:8688/tasks/create
{
"task_type": "compute",
"graph": "testdb",
"params": {
"compute.algorithm": "jaccard",
"compute.parallel":"10",
"compute.max_step":"2",
"jaccard.source":"123",
"output.type":"local",
"output.parallel":"1",
"output.file_path":"result/jaccard"
}
}
3.13 Personalized PageRank
个性化的 pagerank 的目标是要计算所有节点相对于用户 u 的相关度。从用户 u 对应的节点开始游走,每到一个节点都以 1-d 的概率停止游走并从 u 重新开始,或者以 d 的概率继续游走,从当前节点指向的节点中按照均匀分布随机选择一个节点往下游走。用于给定一个起点,计算此起点开始游走的个性化 pagerank 得分。适用于社交推荐等场景。
由于计算需要使用出度,需要在读取图时需要设置 load.use_out_degree 为 1。
request 示例:
POST http://localhost:8688/tasks/create
{
"task_type": "compute",
"graph": "testdb",
"params": {
"compute.algorithm": "ppr",
"compute.parallel":"100",
"compute.max_step":"10",
"ppr.source":"123",
"ppr.damping":"0.85",
"ppr.diff_threshold":"0.00001",
"output.type":"local",
"output.parallel":"1",
"output.file_path":"result/ppr"
}
}
3.14 全图 Kout
计算图的所有节点的k度邻居(不包含自己以及1~k-1度的邻居),由于全图kout算法内存膨胀比较厉害,目前k限制在1和2,另外,全局kout算法支持过滤功能( 参数如:“compute.filter”:“risk_level==1”),在计算第k度的是时候进行过滤条件的判断,符合过滤条件的进入最终结果集,算法最终输出是符合条件的邻居个数。
request 示例:
POST http://localhost:8688/tasks/create
{
"task_type": "compute",
"graph": "testdb",
"params": {
"compute.algorithm": "kout_all",
"compute.parallel":"10",
"output.type":"local",
"output.parallel":"10",
"output.file_path":"result/kout",
"compute.max_step":"2",
"compute.filter":"risk_level==1"
}
}
3.15 集聚系数 clustering coefficient
集聚系数表示一个图中节点聚集程度的系数。在现实的网络中,尤其是在特定的网络中,由于相对高密度连接点的关系,节点总是趋向于建立一组严密的组织关系。集聚系数算法(Cluster Coefficient)用于计算图中节点的聚集程度。本算法为局部集聚系数。局部集聚系数可以测量图中每一个结点附近的集聚程度。
request 示例:
POST http://localhost:8688/tasks/create
{
"task_type": "compute",
"graph": "testdb",
"params": {
"compute.algorithm": "clustering_coefficient",
"compute.parallel":"100",
"compute.max_step":"10",
"output.type":"local",
"output.parallel":"1",
"output.file_path":"result/cc"
}
}
3.16 SCC(强连通分量)
在有向图的数学理论中,如果一个图的每一个顶点都可从该图其他任意一点到达,则称该图是强连通的。在任意有向图中能够实现强连通的部分我们称其为强连通分量。它表明各个点之间的连通性,区分不同的连通社区。
POST http://localhost:8688/tasks/create
{
"task_type": "compute",
"graph": "testdb",
"params": {
"compute.algorithm": "scc",
"compute.parallel":"10",
"output.type":"local",
"output.parallel":"1",
"output.file_path":"result/scc",
"compute.max_step":"200"
}
}
🚧, 后续随时更新完善,欢迎随时提出建议和意见。